https://ift.tt/pJis1Hv What makes a strong candidate? Photo by JESHOOTS.COM on Unsplash What should we look for in data science inter...
What makes a strong candidate?
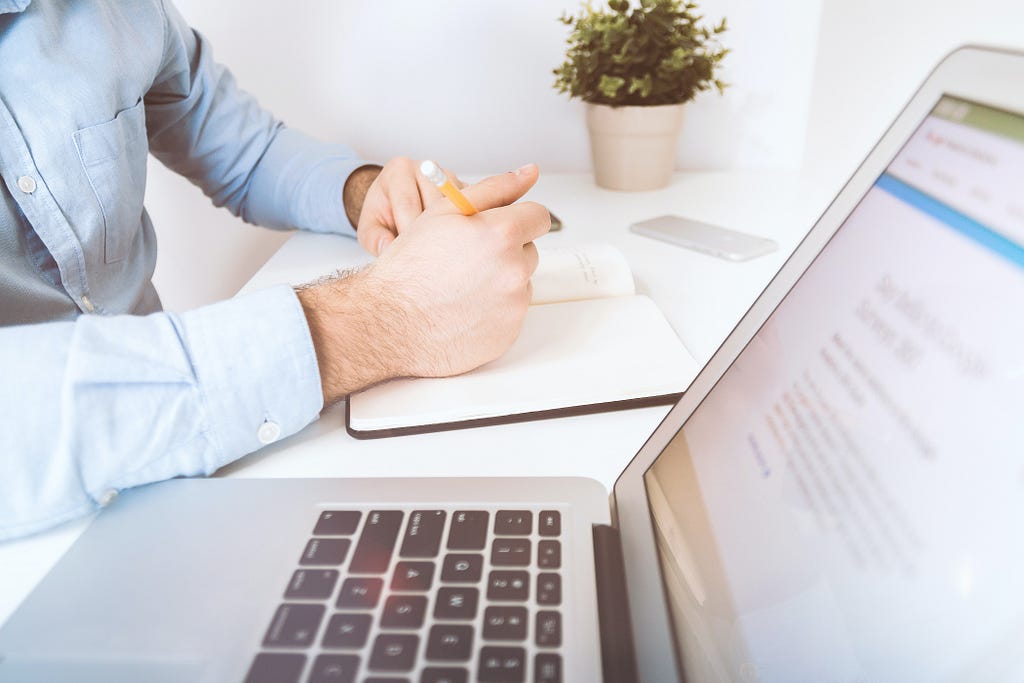
What should we look for in data science interviews? Whether recruiting interns or Sr. Staff, I want to build a team of excellent critical thinkers who can develop data products independently and build a strong rapport with their colleagues. Here I’ll discuss the qualities I look for during data scientist interviews at all levels and how I gauge some of those qualities when meeting candidates for the first time.
A disclaimer before we begin — these qualities are critical in building a team of generalists. That’s partly reflective of my preferences. I believe a healthy amount of context-switching and opportunities for interdisciplinary thinking make work more satisfying and produce more novel solutions.
It’s also what our team and our business need. As our organization matures into a data product company, the opportunity space for data science development grows. Many new questions — about the product, architecture, technical approach, and process — need to be addressed, and we need generalists who can comfortably adapt and apply themselves to new problem spaces. Your mileage may vary depending on your organization and team’s needs, but I’ve found these five qualities critical for candidates to succeed in our context.
Interest in the Role
Though it may sound trite, a strong interest in the role might be one of the chief indicators of long-term satisfaction. We empower our data scientists with wide latitude to spin up and pursue exploratory projects, where a strong sense of curiosity and imagination about the product and user experience is required. Candidates who demonstrate a genuine interest in the problem space are much more likely to be satisfied by the work and bring new creative ideas into the mix.
Our team is also involved in upstream research and development of data products which may take a long time to see the light of day. In an organization developing novel, regulated data products, patience helps see projects through. During such projects, genuine interest in the mission can be a well of energy when the road to success is long.
Data Science Proficiency
Unsurprisingly, we do care about candidates’ data science qualifications. However, as a team of generalists, we rarely hire for specific projects or specialties and are less interested in experience with particular tools, technologies, or techniques. I look for comfort with the fundamentals needed to succeed in the role — table manipulation, hypothesis testing, etc. — but I’m most interested in how candidates approach new problems.
In interactive and collaborative interviews, I prefer to probe a candidate’s problem approach through case studies. What are their first instincts during exploratory data analysis? How do they dispense with edge cases? What is their rationale for selecting one model architecture over another? In their answers, I look for clearly articulated, structured thinking. Candidates should be able to rationalize their design decisions, demonstrate self-awareness of any trade-offs in their approach, and hypothesize how they would mitigate any weaknesses (with additional data, resources, experiments, etc.).
Product Sense
Our team is a central data science function that supports business units without analytics resources and embeds data scientists within product teams to research and develop new features. Often serving as the data experts on cross-functional teams, success in the role requires more than technical mastery and domain knowledge. Our scientists need to interpret how to leverage the organization’s data to address customer needs, whether those customers are our product users or internal business stakeholders.
Like data science proficiency, I prefer case studies to evaluate how candidates think through hypothetical use cases. The primary goals during these exercises are clearly articulating a structured approach to identifying stakeholder needs, proposing technical solutions, and assessing success or impact. How do they approach defining metrics? What is the impact on end users? Do they position our data products as one component of the holistic user experience? The strongest candidates foreground the user experience and holistic impact of data products over their technical performance.
Communication Ability
Because our data scientists are often the primary liaison for all data-related inquiries in cross-functional teams, we usually play the role of data interpreters. Our team is responsible for translating user needs to data product requirements, developing and reviewing those products with technical stakeholders and peers, and translating the results into reports or presentations for business stakeholders.
The ability to communicate clearly and efficiently with technical and non-technical stakeholders is a critical skill and key criterion in our interview process. While we don’t employ any take-home component in our technical interviews, I ask candidates to give a short presentation to a cross-functional group. Listening to a candidate present their work allows other data scientists and business folks to gauge how a candidate speaks to diverse audiences, uses data visualizations, and tells stories with data.
Collaboration Approach
Our data scientists don’t work in a silo; witnessing their communication style is as important as understanding how someone works alongside others. We are a globally-distributed team within a diverse company, and we value inclusivity in communication and collaboration. We reflect that culture in our hiring process, where we look for folks eager to collaborate within an equity-focused culture.
Collaboration experience encompasses work with other data scientists and technical contributors, as well as with business stakeholders or product teams. I want to understand a candidate’s experience contributing to shared codebases, pair programming, and performing technical reviews, as well as their approach to bringing together stakeholders, clarifying roles and responsibilities, and managing conflict resolution. The more senior the position, the greater weight I’ll assign to these skills. Our senior scientists spend more time as technical project leaders, responsible for managing cross-functional teams where a well-developed ability to collaborate and influence is critical.
Final Thoughts
One aspect of our process I didn’t spend much time discussing was technical screening. Early in our interview funnel, we engage candidates in table manipulation exercises, but I don’t prefer spending much energy searching for specific toolkits or technical backgrounds. As a team of generalists, I believe in empowering data scientists with plenty of time to perform literature reviews, learn new skills, and test new techniques. Whatever your background, if you have the qualities described above, we will find the time to acclimate to any relevant tools or technologies once you’re on the team. Equipping teams with the tools and time they need to succeed is a core tenet of my management philosophy and assumed as part of our interview process.
These guiding principles have guided me in honing our interview process and building a high-impact data science function. I hope this guide helps you prepare for your next interview on either side of the table.
The views expressed within are my personal opinions and do not represent the opinions of any organizations, their affiliates, or employees.
Five Skills to Crack Data Science Interviews was originally published in Towards Data Science on Medium, where people are continuing the conversation by highlighting and responding to this story.
from Towards Data Science - Medium
https://towardsdatascience.com/five-skills-to-crack-data-science-interviews-b1ec2d73dedb?source=rss----7f60cf5620c9---4
via RiYo Analytics
ليست هناك تعليقات