https://ift.tt/dqV8AIX Relegating all data consumption to self-service is not as effective as we’d hoped There was a period in the 80s-90s...
Relegating all data consumption to self-service is not as effective as we’d hoped
There was a period in the 80s-90s in the U.S. where the world collectively dreamt of building increasingly complex car dashboards. I remember ogling Christopher Lloyd’s time machine DeLorean in Back to the Future, and I think I (and the rest of the world) subconsciously internalized that a simple car dashboard could do anything, provided we added enough screens and toggles.
But [unfortunately for the 8 year olds in all of us], the dashboard never materialized into the end-all solution to navigational needs that we’d all hoped for. We now generally leverage two vastly different sources of data when driving: the dashboard, certainly, but also the phone. While dashboards help us assess the state of our cars in transit (how’s our car doing?), phones are uniquely better at helping us decide where to go and how we’ll get there. Our navigational imperative overrode our 80s dreams, and our methods of automotive data ingestion have duly bifurcated.
Corporate decision-making is a lot like driving. Strong businesses similarly leverage data in service of answering the same two questions answered by our dashboard and phone: how are we doing (dashboard), and where do we go (the phone)? And analytics teams are responsible for supplying and interpreting the metaphorical phone and dashboard to those ends, which are, respectively:
- Ad hoc analytics: Work that directly answers questions.
Examples: one-off work, data pull requests, stakeholder questions. - Self-service analytics: Work that makes systems to answer questions.
Examples: dashboards, data apps.
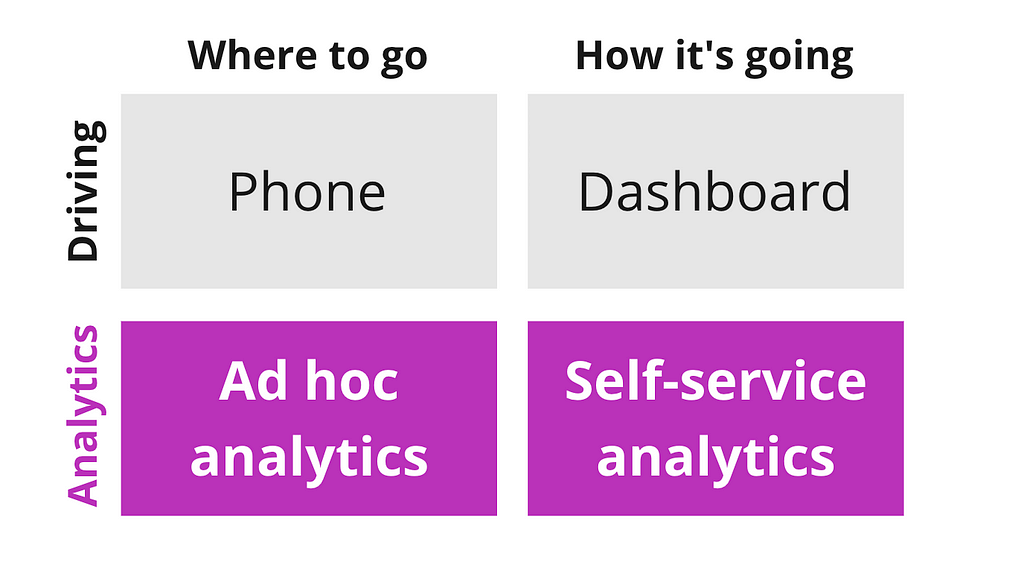
But like our 80s and 90s inner children (and perhaps subconsciously following their cues), the data industry has been chasing the fallacy that increasingly complex dashboards are universal remedies to our decision-making needs, leading to a flood of unmaintained dashboards reminiscent of futuristic 80s car design. But just as when driving, you need data to inform both where to go and how you’re doing to make good decisions as an organization, and thus, both ad hoc and self-service analytics are key to enabling organizations to make data-driven decisions.
Self-service work empowers stakeholders to do basic explorations themselves, while ad hoc analyses help them answer the more sophisticated questions that will inevitably follow.
In what follows, I’ll elaborate on why ad hoc analytics needs to be a first-class citizen, and we’ll discuss what we can do to that end.
Why should you care?
There are three primary reasons why ad hoc work needs to be elevated:
1. Ad hoc analyses are critical to making data-driven decisions
We drove without phones for a long time, but it’s hard to imagine deciding where to go and how to get there without one these days. Are we to use paper maps? Ask our friends for recommendations? How archaic!
Ad hoc analyses are like phones. They help us navigate the world of corporate decision-making by enabling the most critical questions to be answered: “which acquisition channel should we invest in?”, “how do we evaluate the success of this feature without an A/B test?” This class of questions is often not answerable through a dashboard alone, but only through careful ad hoc analysis, deep collaboration with stakeholders, and expert analyst framing and interpretation.
2. Ad hoc work can be the most painful — or most rewarding — part of analytics work
Not all ad hoc work is created the same. A seldom discussed point in analytics circles: there are two distinct kinds of ad hoc requests: strategic analyses and quick questions. While quick questions are requests for data and data alone, strategic analyses are requests for interpretation. Heavy influxes of quick questions can lead to low job satisfaction, with analysts being treated like SQL monkeys, while strategic analytics work can lead to high job satisfaction, with analysts being included in conversations as valuable thought partners. The trick here: behind each quick question, there’s always a strategic request.

Building processes and best practices for analytics teams to intentionally uncover the strategic questions underneath data pull requests can lead to substantially improved job satisfaction. But this only comes when ad hoc work is treated as a legitimate workflow, not swept under the rug.
3. Ad hoc work takes up a lot of time
We polled folks on Twitter, LinkedIn, and in person, and found that around 40-50% of analyst time is spent on ad hoc requests, even with robust tooling to enable self-service behavior (Looker, metabase, dbt, etc.). Ad hoc work is not only painful, it takes up a ton of time.
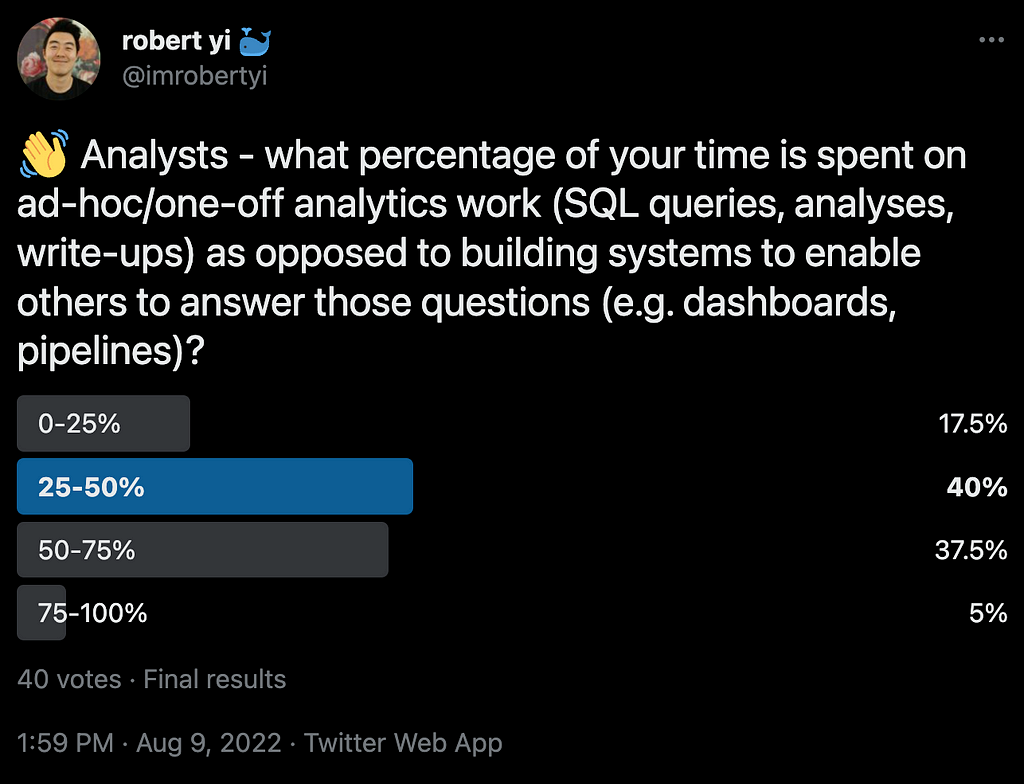
What can you do about it?
1. Make ad hoc work a first class citizen.
Simply recognizing that ad hoc work is valid work can go a long way. For team leads, ensure that ad hoc work is properly incorporated into performance reviews for analysts. For ICs, learn how to do these effectively: avoid reactively, thoughtlessly responding to requests, but working with stakeholders to involve yourself in uncovering the underlying strategic objective before you write a single line of SQL. And for stakeholders, learn to ask questions that involve analysts more deeply into the decision-making process.
2. Establish a system and standards for ad hoc work.
Ad hoc work deserves process and standardization. Build templates for analysts to ensure work is standardized, nudge stakeholders to follow their own processes to streamline conversations, store work in a central, discoverable place. We’ve built hyperquery precisely to make it easier to do and share strategic ad hoc work, but the objective is the process improvement, not the tool.
Conclusion
While the self-service dashboarding world is certainly preferable to the data desert of generations past, there’s a lot of work to be done, and relegating all data consumption to self-service is not as effective as we’d hoped. A strategy for ad hoc work needs to be a key part of any robust analytics plan.
Interested in learning more about super-powering your ad hoc workflows? Reach out to me at robert@hyperquery.ai or schedule a call with us, and check out our dedicated platform for ad hoc analytics at hyperquery.ai.
What Is Ad Hoc Analytics, and Why Should You Care? was originally published in Towards Data Science on Medium, where people are continuing the conversation by highlighting and responding to this story.
from Towards Data Science - Medium https://ift.tt/V83m6Ut
via RiYo Analytics
ليست هناك تعليقات