https://ift.tt/0AJOQIo Data teams are more important than ever before — but they need to get closer to the business. Here’s how we can righ...
Data teams are more important than ever before — but they need to get closer to the business. Here’s how we can right the ship.
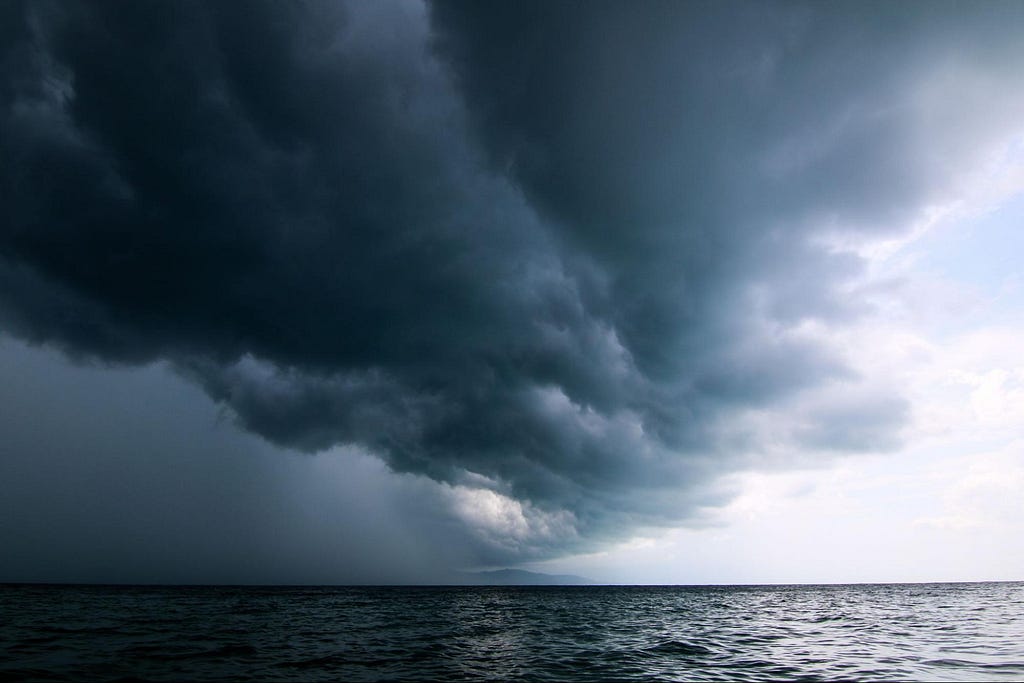
Over the past decade, data teams have been simultaneously underwater and riding a wave.
We’ve been building modern data stacks, migrating to Snowflake like our lives depended on it, investing in headless BI, and growing our teams faster than you can say reverse ETL. Yet, much of the time we didn’t know whether or not these tools are actually bringing value to the business.
Don’t get me wrong: I’m a firm believer in the modern data stack. Cloud-native, SQL-based, and modular is the way to go when it comes to generating analytics quickly and efficiently. But in today’s day and age of tight budgets, lean teams, citing the elasticity and speed of the cloud isn’t enough to justify the investment in these tools.
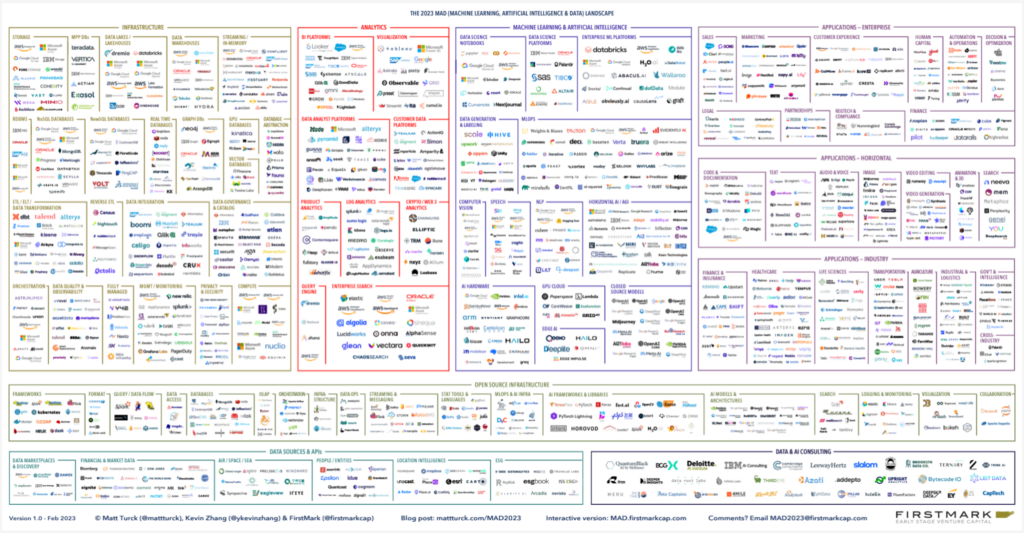
Now when the waiter drops off the check, rather than plopping down the credit card without a second thought, the CFO is investigating each line item.
For data teams, the bill has come due. What should be an opportunity has become a crisis as organizations discover they aren’t close enough to the business to explain why they ordered the lobster.
How did we get here?
Data teams made history as one of the first departments to spin up 8-figure technology stacks with little to no questions asked.
In the mid-2010s, data leaders were tasked with “becoming data-driven” — whatever that meant. Of course, data can help optimize costs, improve financial performance, guide product roadmaps, deliver amazing customer insights, and gain competitive advantage. But becoming “data-driven” is an opaque goal with vague metrics and no clear ROI.
Of course, data teams didn’t go rogue. The train was moving full steam ahead in the “growth at all costs” era. Executives and decision makers looked at who was winning–the Googles, Netflixes, and Amazons of the world–and investing in data seemed like a no brainer.
Our nascent industry built stacks hacked together internally or purchased ad hoc to solve specific problems. Whether or not these systems — and the data itself — integrated with expected, software engineering-inspired five nines of reliability was often an afterthought.
Simply having data at this stage was often enough to fuel growth. Some data was useful, some data wasn’t, but we at least had it. By 2020, the pandemic added more fuel to the fire as everything became digital and started emitting data.
Technologies like Snowflake, Databricks, and Fivetran showed up like magic to solve many of the problems associated with “becoming data-driven.” Faster insights? Check! Easier ingestion? Check! Smarter models? Check!
Eventually, however, these solutions started tying volume of data to cost. Fast forward to today, your data is growing rapidly every year and you’re looking at 1000x the data volume and 1000x the cost. In this market, that’s a tough pill to swallow.
To make it out ahead and justify our work, providing data isn’t enough. It also needs to be reliable and purpose built.
In other words, data teams need to get closer to the business.
So how do we get there? I have a few thoughts.
Get to know the business by getting to know the people
Have you ever bought a gift for someone you haven’t seen in years, for instance, an old childhood friend or distant cousin, based on one random fact you know about them (i.e., they love penguins)?
If the answer is yes, you’re definitely not alone. Data teams, like a distant friend gifting a seemingly thoughtful present, want to do right by their stakeholders, with rich insights that can improve their work and bring value to the business. But you can’t fake data empathy.
If you don’t intimately understand consumer needs, your reports and analysis will be about as valuable as a 5-foot stuffed penguin.
The first thing data leaders should do when it comes to driving value is talk to their consumers and business stakeholders. This is obvious advice, yet the task of “requirement gathering” is often delegated to analysts or embedded teams. That works up to a point, as anyone who has played a game of telephone can tell you.
For example, Red Ventures director of data engineering, Brandon Beidel, met with every business team in a weekly cadence to better understand their use cases and create informed data SLAs.
He said, “I would always frame the conversation in simple business terms…I’d ask:
- How do you use this table?
- When do you look at this data? When do you report this data? Does this data need to be up to the minute, hourly, daily?
- What purpose does this serve?
- Who needs to get notified if this data is delayed?”
An advantage to this type of hands-on leadership? You can shape the engagement.
“I would also push back if I was told the data was important but no one could tell me how it was used. Conversations became more sophisticated and I would even get descriptions that could quickly transform into a query like, ‘no null values in this column,’” Brandon said.
You can also survey your data consumers en masse similar to how product teams launch NPS surveys, which is a strategy JetBlue’s data team discussed during a recent webinar.
Create asynchronous feedback loops
You can’t always talk to everyone live all the time. Asynchronous communication and feedback loops are essential for data, business alignment (especially in today’s remote world).
If you don’t have a widely accessible and active Slack channel for these types of communications, consider creating this type of communication space immediately. This was one of the keys to how director of data and analytics, Priya Gupta, created data culture at hypergrowth startup Cribl. She says:
“Our data team errs on the side of overcommunication, and we try to communicate across as many channels as possible. Just like we as data engineers look askance at a data source that isn’t well documented, business users are going to be inherently skeptical towards quiet data teams.
Chat applications like Slack or Teams are very helpful in this regard. We create a centralized channel for all requests, which serves two purposes. It gives the entire analytics team visibility into requests, and it allows for other stakeholders to see what their peers are interested in.
The most satisfying thing you’ll ever see is when someone makes a request in your public Slack channel and then another stakeholder answers the question that was meant for you.”
But perhaps the most important feedback loop is how you surface the trustworthiness and reliability of your data products to your consumers. Not all data products will be, or need to be, 100% reliable.
Once you’ve created a mechanism for self-service or discovery, go the extra mile to display both the reliability SLA as well as the percentage of time the product meets that SLA. This is exactly the strategy Roche takes when building data products on their data mesh.
Walk a mile in your data consumers’ shoes
If you are feeling ambitious, the next step is to move from talking the talk to walking the walk. After all, business stakeholders aren’t always familiar with the art of the possible when it comes to data.
Similar to how Henry Ford said the consumer would have asked him for a faster horse, sometimes the data consumer is just going to ask for a faster dashboard. Instead of looking to business stakeholders to tell us what matters, we need to start generating our own hypotheses on where to look, what to look for, and how to apply these insights.
To do this, have a member of the data team get in the dirt with stakeholders and live their life with data.
That is the strategy of the analytics engineering team at Upside developed after senior data analytics engineer Jack Willis “… realized that a lot of the [data] products made for this team didn’t hold up to snuff [when] I actually saw what they were doing with it.”
Their data enablement framework involves three steps: fully embed within the team, plan alongside stakeholders, and train teams to ensure sustainable ownership. It is an effective way to maximize the value of newer roles like analytics engineers who can bridge the gap between data engineers and analysts. They can come to the table with greater insight into how the business works and what data will actually move the needle.
“We build a pathway of trust in the data products…where our stakeholders aren’t afraid of data and engineering and our data practitioners aren’t afraid of the business …This puts us on a data flywheel,” said Jack.
Let adoption be your guide
You don’t always need to live the data consumer’s life to understand their story. It’s also told through what they adopt…and what they don’t. In fact, “dark data” and data silos can be more informative than well-adopted data products.
V_GOOD_DASHBOARD_48 is more valuable than V_GOOD_DASHBOARD_49 if it’s the one being exported to Excel by your business operations team (even if it pains you to know that your Looker skills are undervalued).
Before you transition from your customer data platform to a more cloud-native solution, understand how the marketing team uses it and why. Just as much value may derive from intuitive self-service access as the powerful customer segmentation you can provide.
To do this, data teams need to invest in approaches and technologies that surface who is using what data and how.
But modern data stacks in and of themselves don’t give data teams greater visibility into their data, nor does it increase transparency between data teams and stakeholders. If anything, the modern data stack can complicate this already tenuous relationship by giving greater access with less clarity and context.
We need ways to draw connections between upstream tables and downstream assets in a way that incorporates the entirety of your data environment — not just the warehouse, lake, or transformation layer. We need an approach that’s truly end to end, all the way down to the consumption layer.
In today’s day and age, ignorance isn’t bliss; it’s a recipe for failure. Visibility and transparency into our data assets will help us prioritize, stay focused, and actually move the needle for the business.
Create a semantic layer
Our industry is making progress on codifying the semantic layer, and self-serve data platforms are putting more power in the hands of analysts to get their hands dirty with the data in ways they haven’t before.
It is virtually impossible to create a semantic layer, sometimes referred to as a metrics layer, without having deep conversations about how the business thinks about and uses data.
As you are creating the one universal truth for what an “account” means and talking to business stakeholders to determine if that should include freemium users, you are deep in the weeds exploring and cementing the true drivers of your business.
A semantic layer can be a great excuse to hold the conversations you never had on points that you should seem to know. Your C-suite probably feels the same way. They don’t really understand what an “account” means either.
As a true semantic layer begins to form, solutions that help you develop and standardize your north-star metrics as augmented analytics platforms or data notebooks — can provide a nice stop-gap.
Focus on short-term wins that matter
Fielding ad-hoc requests, investigating broken data pipelines, and responding to Bob from Finance’s fifth question of the day are all quick wins, but they don’t meaningfully move the needle.
On the other hand, company-wide initiatives launched with multi-year time horizons are often doomed prior to the kick-off call. Big “Capital P” Projects (data mesh, anyone?) are important and worthwhile, but they don’t need to be “complete” to be useful. The best bet is to focus on small, short-term wins with clear business value.
Again, adoption should be your guide. Focus the majority of your resources on optimizing and building upon your key use cases and data assets (you DO know your key data assets right?).
You want to improve data trust? Focus on a small subset of your data that correlates with the highest impact, such as customer behavior or third-party marketing data.
- Segment data by domains so you know what leg of the business is responsible when something breaks
- Spend as little time as possible on freshness, volume, and schema checks (you know, the easy ones) and then focus on writing custom rules to catch distribution or field health anomalies.
- Spin up Slack, Teams, or carrier pigeon channels between data teams and business stakeholders to alert all relevant parties when data breaks and, with the assistance of lineage, advise on what reports and dashboards have been impacted.
Does narrowing your focus mean you won’t make everyone happy. Yes. Does narrowing your focus mean you’ll be more successful this quarter? Yes.
Crisis averted?
Solving our next big crisis won’t happen overnight. Like any type of change, bringing our work closer to the business will take time, ruthless prioritization, and the ability to understand where we can drive the most value.
Personally, I couldn’t be more excited for what the future holds as we adopt more processes, technologies, and team structures that take data teams out of the storm and into calmer waters.
So, how is your team going to navigate our next big crisis? I’m all ears.
Reach out to Barr on LinkedIn to air your frustrations, send data memes, or share your experiences navigating this crisis. Always happy to connect.
The Next Big Crisis for Data Teams was originally published in Towards Data Science on Medium, where people are continuing the conversation by highlighting and responding to this story.
from Towards Data Science - Medium
https://towardsdatascience.com/the-next-big-crisis-for-data-teams-58ac2bd856e8?source=rss----7f60cf5620c9---4
via RiYo Analytics
No comments