https://ift.tt/V4gqj29 Opinion ChatGPT may have consumed as much electricity as 175,000 people in January 2023. Image: Midjourney I re...
Opinion
ChatGPT may have consumed as much electricity as 175,000 people in January 2023.
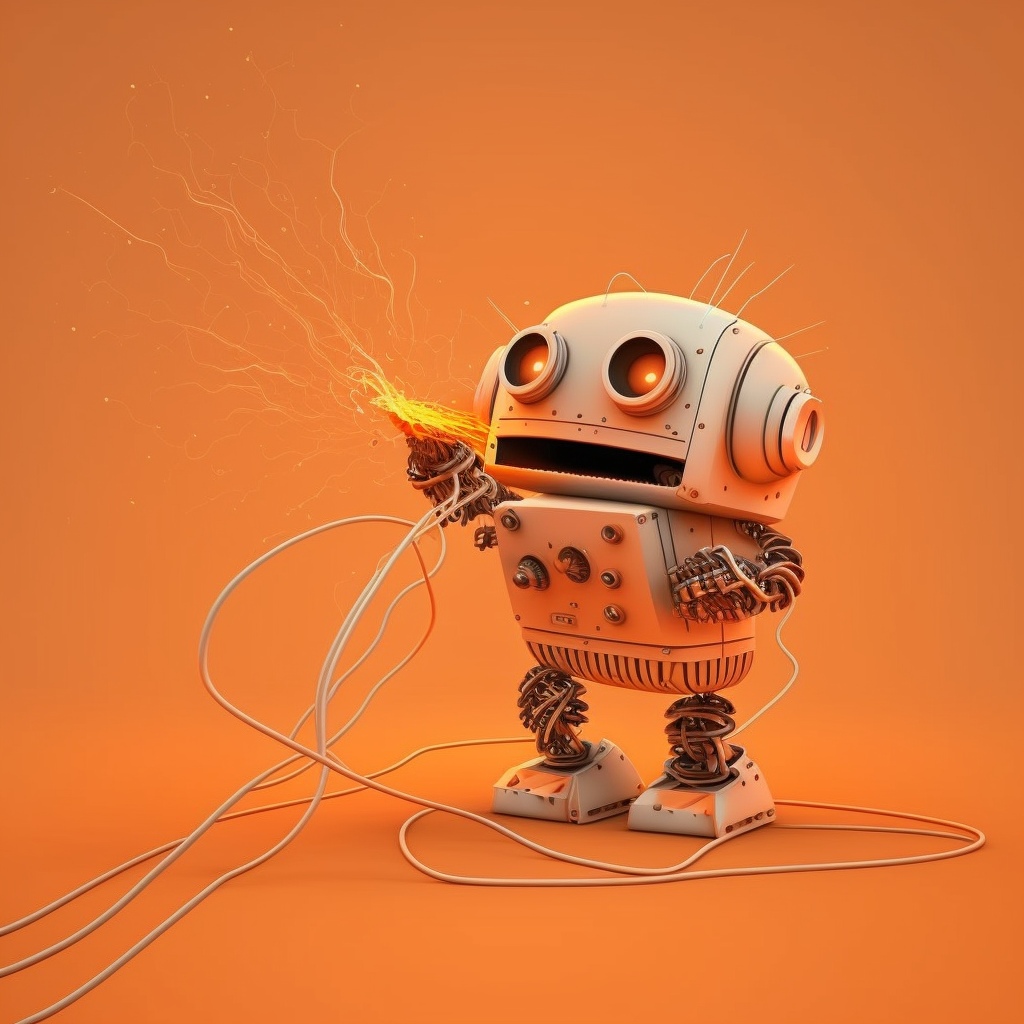
I recently wrote an article in which I guesstimated ChatGPT’s daily carbon footprint to the around 24 kgCO2e. With little information available at the time about ChatGPT’s user base, my estimate was based on the assumption that the ChatGPT service was running on 16 A100 GPUs. In lieu of recent reports that estimate that ChatGPT had 590 million visits in January [1], it’s likely that ChatGPT requires way more GPUs to servce its users.
From this it also follows naturally that ChatGPT is probably deployed in multiple geographic locations. This makes it very difficult to estimate ChatGPT’s total daily carbon footprint, because we would need to know exactly how many GPUs are running in which regions in order to incorporate the carbon intensity of electricity in each region into the carbon footprint estimate.
Estimating ChatGPT’s electricity consumption, on the other hand, is in principle simpler, because we do not need to know in which geographic regions ChatGPT is running. Below I explain how one can go about estimating ChatGPT’s energy consumption and I specifically produce an estimate of ChatGPT’s electricity use in January 2023. The scope is limited to January 2023 because we have some ChatGPT traffic estimates for this month.
The Carbon Footprint of ChatGPT
Estimating ChatGPT’s electricity consumption
Here’s how ChatGPT’s electricity consumption can be estimated:
- Estimate ChatGPT’s electricity consumption per query
- Estimate ChatGPT’s total number of queries for a given period in time
- Multiply those two
To calculate a range within which ChatGPT’s electricity consumption may lie, I’ll define 3 different values for each of points 1 and 2. Let’s first take a look at point 1.
Step 1: ChatGPT’s electricity consumption per query
BLOOM is a language model similar in size to ChatGPT’s underlying language model, GPT-3.
BLOOM once consumed 914 KWh of electricity during an 18 day period where it was deployed on 16 Nvidia A100 40 GB GPUs and handled an average of 558 requests per hour for a total of 230,768 queries. Queries were not batched. The 914 KWh account for CPU, RAM and GPU usage [2].
So BLOOM’s electricity consumption in that period was 0,00396 KWh per query.
Let’s consider how good a proxy BLOOM’s electricity usage might be for ChatGPT’s electricity usage.
First things first: BLOOM has 176b parameters, and GPT-3 (ChatGPT’s underlying model) has 175b parameters, so everything else held equal, their energy consumption should be quite similar.
Now, a few things suggest that ChatGPT’s electricity consumption per query could be lower than BLOOM’s. For instance, ChatGPT receives way more requests per hour than BLOOM did, which could lead to a lower energy consumption per query. Figure 1 below plots BLOOM’s electricity use against the number of requests and we can see that even at 0 requests, BLOOM had an idle consumption of 0.3 KWh, and at 1,000 requests the electricity consumption was less than 5 times higher than at 200 requests.
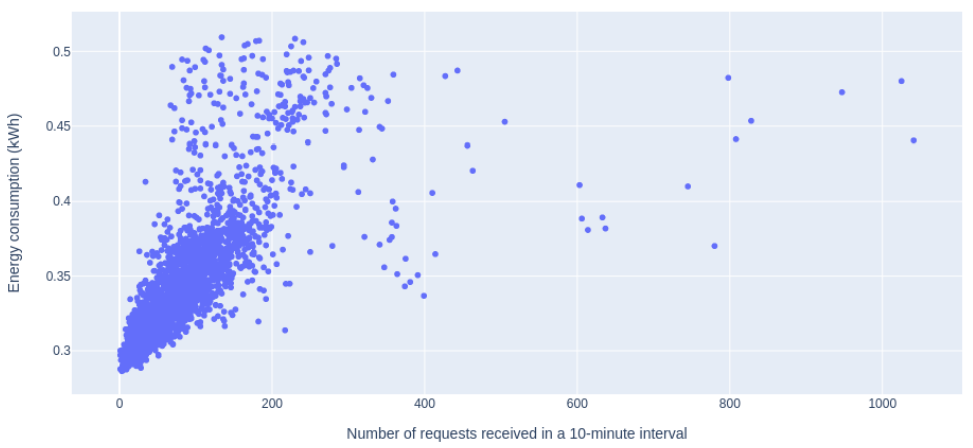
Although the authors of the paper that measured BLOOM’s electricity consumption state that “since inference requests are unpredictable, optimization of GPU memory using techniques such as batching and padding […] is not possible” ([2] p. 5), we might expect that ChatGPT experiences a constant rate of requests high enough to make batching feasible. I would guess that batching of queries would lead to a lower energy consumption per query.
One thing that could increase ChatGPT’s energy consumption per query is if ChatGPT on average generates more words per query, but we don’t have access to any information that let’s us approximate this.
To err on the side of caution, I’ll assume in the following that ChatGPT’s electricity consumption is at max the same as BLOOM’s, so I’ll make an estimate of ChatGPT’s electricity consumption for each of the following assumptions:
- ChatGPT’s electricity consumption per query is the same as BLOOM’s, i.e. 0.00396 KWh
- ChatGPT’s electricity consumption per query is 75 % of BLOOM’s, i.e. 0.00297 KWh
- ChatGPT’s electricity consumption per query is 50 % of BLOOM’s, i.e. 0.00198 KWh
How to estimate and reduce the carbon footprint of machine learning models
Step 2: Number of queries sent to ChatGPT
Now that we have some estimates of ChatGPT’s electricity consumption per query, we need to estimate how many queries ChatGPT received in January.
It’s been estimated that ChatGPT had 590m visits in January 2023 [1]. How many queries did each visit result in? We don’t know, but let’s make some assumptions. I would expect that each visit results in at least 1 query, so that’s the minimum assumption. Then, I’ll make a scenario with 5 and 10 queries per visit. Annecdotally, I’ve heard several people report that they use ChatGPT extensively in their daily tasks, so I believe these numbers are not unreasonable.
- Queries per visit = 1, so total number of queries = 590m
- Queries per visit = 5, so total number of queries = 2.95b
- Queries per visit = 10, so total number of queries = 5.9b
# 3 : Combining electricity consumption estimates with number of queries
Above, I outlined three different assumptions regarding ChatGPT’s electricity use per query and three different assumptions regarding the total number of queries.
This gives us 9 unique set of assumptions that I will refer to as “scenarios”.
Table 1 below shows the estimated electricity consumption for each of the scenarios.
The table shows that ChatGPT’s electricity consumption in January 2023 is estimated to be between 1,168,200 KWh and 23,364,000 KWh.
Here’s an online spreadsheet if you want to check my calculations.
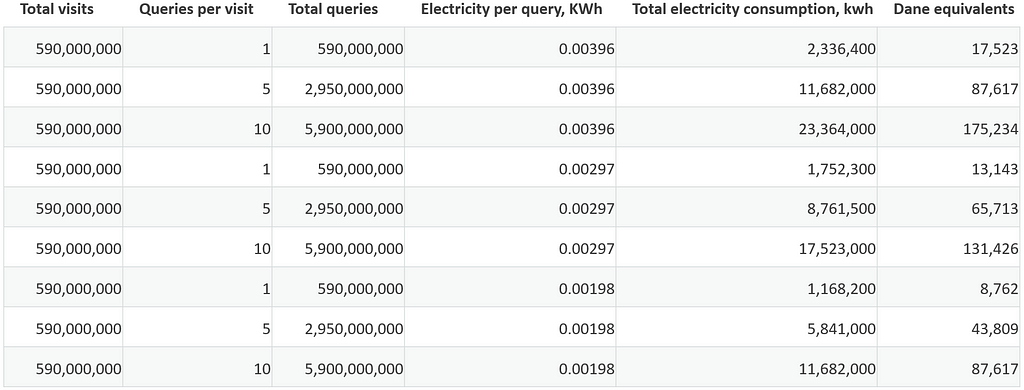
ChatGPT may have used more electricity than 175,000 Danes
Let’s put the numbers from Table 1 into perspective.
The annual electricity consumption of the average Dane is 1,600 KWh [3].
If the annual consumption is spread out evenly over the year, the average Dane used about 133.33 KWh of electricity in January.
So when we divide ChatGPT’s estimated electricity consumption by 133.3, we can see ChatGPT’s estimated consumption correspond to the monthly electricity consumption of between 8,762 and 175,234 Danes.
To put it into another perspective, you can run a standard 7w light bulb for around 19,050 years with 1,168,200 KWh of electricity (1,168,200 KWh / (7 / 1000) / 24 / 365).
Training ChatGPT’s underlying language model, GPT-3, consumed an estimated 1,287 MWh [4].
So at the low end of the estimated range for ChatGPT’s electricity consumption in January 2023, training GPT-3 and running ChatGPT for one month took roughly the same amount of energy. At the high end of the range, running ChatGPT took 18 times the energy it took to train.
Conclusion
Although handling a single query requires miniscule resources, the total amount of resources (e.g. electricity) add up to noticeable amounts when a product like ChatGPT scales. The search engine Bing now has incorporated ChatGPT [5] which might significantly increase the number of queries ChatGPT should handle, thereby also increasing the electricity consumption. In my opinion, this requires a debate about whether the incorporation of large machine learning models into existing products elevate the user experience to a level that justifies the large energy consumption.
In addition, unless the estimates put forward here are way too high, this article shows that running a machine learning based product in production can easily be way more energy consuming than training the model — especially if the product has a long life time and/or a large number of users. This should be taken into consideration when debating the environmental impact of AI and when thinking of how to reduce the life cycle carbon footprint of machine learning models.
It is important for me to emphasize that the estimates put forward in this article come with great uncertainties as very little information about how ChatGPT is run is publicly available. In the best of all worlds, OpenAI and Microsoft — and other tech companies for that matter — would disclose the energy consumption and carbon footprint of their products. But while we wait for that to happen, I hope my estimates will spur a debate and inspire others to come up with better estimates.
That’s it! I hope you enjoyed this post 🤞
I’d love to hear from you:
1) what you think about the analysis and
2) whether you think ChatGPT is worth its electricity consumption.
Follow for more posts related to sustainable data science. I also write about time series forecasting like here or here.
Also, make sure to check out the Danish Data Science Community’s Sustainable Data Science guide for more resources on sustainable data science and the environmental impact of machine learning.
And feel free to connect with me on LinkedIn.
References
[2] https://arxiv.org/pdf/2211.02001.pdf
[4] https://arxiv.org/ftp/arxiv/papers/2204/2204.05149.pdf
[5] https://www.digitaltrends.com/computing/how-to-use-microsoft-chatgpt-bing-edge/
ChatGPT’s electricity consumption was originally published in Towards Data Science on Medium, where people are continuing the conversation by highlighting and responding to this story.
from Towards Data Science - Medium
https://towardsdatascience.com/chatgpts-electricity-consumption-7873483feac4?source=rss----7f60cf5620c9---4
via RiYo Analytics
ليست هناك تعليقات