https://ift.tt/KcBHgfQ Healthcare Data Is Inherently Biased Here’s how to not get duped by the data Normally when you think of bias, you ...
Healthcare Data Is Inherently Biased
Here’s how to not get duped by the data
Normally when you think of bias, you might think about a person’s beliefs that inadvertently shape their assumptions and approach. This is certainly one definition.
But bias also refers to how the very data we use for insights can be unsuspectingly skewed and incomplete, distorting the lens through which we see everything.
Many — if not most — of the datasets we use in healthcare are inherently biased and could easily lead us astray if we aren’t careful.
I’ll specifically focus on three major concepts afflicting healthcare data and that may even be low-key invalidating your entire analysis.
In an earlier article, I wrote about the types of bias I’ve encountered in my healthcare analytics career and how they are a challenge for data practitioners in the field. The biggest themes for me being: personal bias, data bias, and confirmation bias.
Four days after I published that article, a mentor sent me this JAMA article about sampling bias in healthcare claims data and how it is exacerbated by social determinants of health (SDOH) in certain regions.
The article’s conclusion really stood out to me:
[The study highlights] the importance of investigating sampling heterogeneity of large health care claims data to evaluate how sampling bias might compromise the accuracy and generalizability of results.
Importantly, investigating these biases or accurately reweighting the data will require data external data sources outside of the claims database itself.
TLDR:
Imbalance of patients or members represented in large healthcare datasets can make your results non-generalizable or (in some cases) flat out invalid.
SDOH data could help.
Let’s unpack this.
But first, a quote from the brilliant Cassie Kozyrkov: “the AI bias trouble starts — but doesn’t end — with definition. ‘Bias’ is an overloaded term which means remarkably different things in different contexts.” Read more about it in her article with lots of other breadcrumbs to related articles, “What is bias?”
As healthcare analysts, we need to be on the lookout for:
1. Sampling/Selection Bias.
Sampling bias is when some members of a population are systematically more likely to be selected in a sample than others. Selection bias can be introduced via the methods used to select the [study] population of interest, the sampling methods, or the recruitment of participants (aka, flawed design in how you select what you’re including). [1]
I’m using the terms somewhat colloquially. The definition of Sampling/Selection bias makes sense in the traditional survey/sample/research design.
But.. red flag #1: “Population” means something VERY specific in data, and equally as specific (but different) in healthcare, and they might be the same or they might not, depending on the thing.
In the context of healthcare, they can refer to how construct our analyses and our inclusions/exclusions to understand a certain “population.” In healthcare, we use populations of interest to refer to many different groups of interest depending on the study, for instance:
- Lines of business (LOB) such as patients with coverage from a government payer (Medicaid, Medicare); commercial lines (employer groups, retail or purchased via the exchange); self-insured (self-funded groups, usually large employers paying for their own healthcare claims of employees), etc.
- Sub-lines of business, or groups. For instance, Medicaid may consist of multiple sub-groups representing different levels of eligibility, coverage, benefits, or types of people/why they qualified for the program (Temporary Assistance for Needy Families (TANF) vs. Medicaid expansion for adults)
- Demographics (certain groups, males or females, certain regions, etc.)
- Conditions (examining certain chronic conditions, top disease states of interest or those driving the highest costs to the system, those that Medicare focuses on, etc.)
- Sub-groups of some combination of the above, for instance: Medicaid, TANF, looking at mothers vs. newborns
- Cross-group comparison of some combination of the above, for instance: Medicaid, TANF, new mothers and their cost/utilization/outcomes trends vs. Commercial, self-insured, new mothers and their cost/utilization/outcomes
- Any sub- or cross-combination of the above, plus a lot more
As you can see, design of the analysis and the “population” in question can get complex very quickly. This doesn’t necessarily mean that the data is not usable or that results will always be dicey. It does reaffirm that being aware of this kind of bias is paramount to ensuring you’re considering all angles and analyzing accordingly.
Flag #2: If someone in healthcare says the data contains the entire population of interest… does it, really? Maybe...
A slight caveat for health insurers: one common talking point is that a health insurer can analyze their “entire population” because they receive claims for every member in their charge, and they can avoid sampling fallacies. This could potentially be a sound talking point, depending on the use case. But in general, I’ll caution you to remember that even that data is inherently biased because it: a) only includes any members/patients who actually had an incident/event for which the insurer processed a subsequent claim and b) the data itself tends to over/underrepresent certain groups who are more likely to have chronic health problems, adverse social determinants of health, seek care, be reflective of the type of demographics your organization tends to serve or that you have a larger book of business in, etc. More on that with the article summary, below.
2. Undercoverage Bias
Undercoverage bias occurs when a part of the population is excluded from your sample. [1]
Again, this definition make sense in the traditional survey/sample/research design. In the context of healthcare, it can bite us in a tangible way (wasted money, wasted effort, shame, having no impact or change in outcomes, or… all of the above) if we are not careful.
Aside from the purest definition above, I also think about this in the context of not being able to see anything that happens “outside of your four walls.” We (generally) only have access to the data our organization has generated, which inherently is only part of the entire picture. This is not a deal breaker depending on what kind of analysis you’re doing and why, but another major flag to be aware of. Our [patients / members / employees / residents / etc.] may not act similarly or even represent [all patients / other groups / other types of employer s / other regions / etc.]
Flag #3: your data only shows what your organization has done internally, so it cannot always be used to infer what your competitors’ truths might look like, what your communities’ truths might look like, what happens to patients when they visit a different healthcare provider that is not you, or if any one health plan member’s behavior is anything like another member’s based on personal, regional, societal, occupational, or behavioral differences (data we usually do not have), to name a few. All of this must be considered as you’re seeking conclusions.
As more healthcare organizations are shifting focus to make a broader difference in the communities they serve more holistically (versus on their specific patients), we might be missing a whole ’lotta pieces of the data puzzle—again, data that tells us what is happening “outside our four walls.”
All this said, some of our analyses may not be so reliant on knowing that, per se. Some of this could be addressed by augmenting our own internal data with external data from outside our four walls to fill in more of the picture. Some of us have recognized this for awhile and are working on data sharing/collaboration or tapping into additional feeds such as health information exchange (HIE) or purchased benchmark data to compare our own population to so we can understand how differently ours looks or acts. Bear in mind that those datasets may suffer from these same biases on a broader scale (see that JAMA article, in fact), but all of these are great first steps towards at least understanding and recognizing any underlying “gotchas.”
3. Historical Bias or Systemic biases.
Historical bias occurs when socio-cultural prejudices and beliefs are mirrored into systematic processes [that are then reflected in the data]. [3] Systemic biases result from institutions operating in ways that disadvantage certain groups. [2]
This becomes particularly challenging when data from historically-biased sources are used for data science models or analyses. It is also particularly important when you’re analyzing historically broken systems, such as healthcare.
This is such a hot topic that NIST is developing an AI Risk Management Framework. NIST talks about human and systemic biases in their special publication, Towards a Standard for Identifying and Managing Bias in Artificial Intelligence, which is where this image hails from:
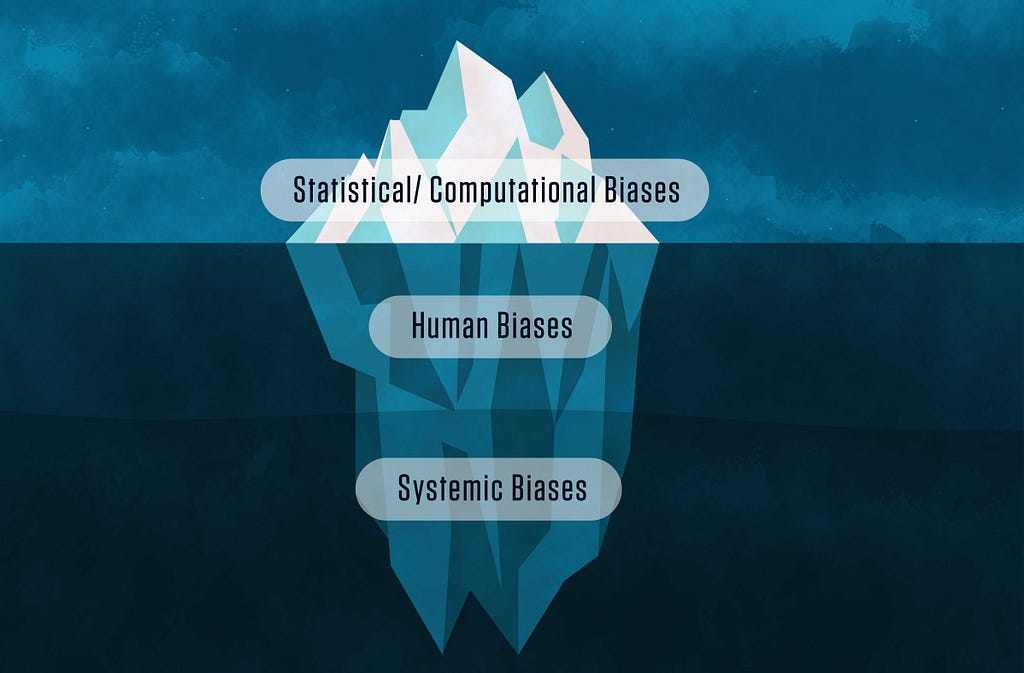
In healthcare, over-and-under-representation of certain diseases (or just sick people in general, because that’s who consumes healthcare), demographics, people of a certain group or sub-group, utilization patterns (or lack thereof), health/quality/mortality/engagement/satisfaction and many other trends or outcomes that we see in our healthcare data are all reflective of the way in which the broken healthcare system operates. This is a highly nuanced topic with a lot of different facets, to be explored in more depth in a later article. But suffice it to say:
Flag #4: The healthcare system is broken and existing societal constructs greatly impact health and outcomes, opportunities (or lack thereof), barriers, and behaviors. This is irrefutably reflected in healthcare data in many different ways.
Cue the Health Equity initiatives.
How can SDOH data help?
This part is not a slam dunk to address everything I’ve outlined, but I thought it was interesting enough to call out specifically as we are talking about Health Equity and SDOH more and more in health analytics lately.
The JAMA article’s authors sought to understand potential bias in large, commercially-available databases comprised of aggregate claims from multiple commercial insurers. These kinds of datasets are commonly used by organizations for clinical research, competitive intelligence, and even benchmarking. The authors analyzed one common such set — the Optum Clinformatics Data Mart (CDM) — which is derived from several large commercial and Medicare Advantage health plans and licensed commercially for various use cases.
At a zip code level, the authors analyzed the representation of individuals in the CDM as compared to Census estimates and the SDOH variability seen for said zip codes. I will note that the datasets they’re using are from 2018; this is another major issue with most healthcare data you can get your hands on, but for another day.
The article finds:
[Even after adjusting for state-level variation, our fancy pants statistical methods] found that inclusion in CDM was associated with zip codes that had wealthier, older, more educated, and disproportionately White residents.
The socioeconomic and demographic features correlated with overrepresentation in claims data have also been shown to be effect modifiers across a diverse spectrum of health outcomes.
Importantly, investigating these biases or accurately reweighting the data will require data external data sources outside of the claims database itself.
TLDR (again):
The claims data was disproportionately skewed towards representing more educated, affluent, and White patients.
The specific SDOH factors impacting people at the zip code level (and sub-zip) have well-documented influence on differences in health outcomes.
Reweighting/normalizing the data requires additional data beyond just the claims.
Some might call that data “outside your four walls.”
So now what?
Your #1 goal: Awareness.
I’ve solely put forth these ideas as a guide for you to start thinking about any underlying “gotchas.” Start by asking yourself and others questions. Start thinking about it as you’re designing your next analysis. Start making sure you fully understand how and where the results will be used, what the intent is, and listing the risks upfront. Start understanding the different types of bias and how they might impact you. If you want to read about 29 other types of bias, further broken down into more subtypes, check out this helpful knowledge base article on Scribbr about research bias.
Your #2 goal: Do not let this cause analysis paralysis.
While it is important to keep this top of mind, it is even more important that we don’t get so hung up on it that we get analysis paralysis. As analysts, knowing the nuances and then deciding where to draw that line in the sand will arguably be the hardest part of your job: deciding which insights are still valuable or meaningful despite these nuances, and when to lean in to (or away from?) the soundness of the conclusion, will be absolutely paramount to your success and your organization’s success.
Most likely, the outcome will probably land somewhere in the middle, so it will is your goal to understand the nuances, communicate them clearly, and provide directionally appropriate guidance to the extent that you can while being a good steward against potential misinterpretation.
This article highlights a subset of biases that are not unique to healthcare, but are very unique in how they apply in healthcare.
What other types of bias have you encountered in your healthcare or analytics career?
Stefany Goradia is VP of Health Lab at RS21, a data science company with a Health Lab devoted exclusively to healthcare + community.
She has spent her career on the front lines of healthcare analytics and delivering value to internal and external customers. She writes about how to interpret healthcare data, communicate it to stakeholders, and use it to support informed decision making and execution.
Like health data and healthcare analytics?
Follow me on Medium and LinkedIn
Like my style?
Learn more about my background and passion for data at stefanygoradia.bio
Some other good reference articles about bias:
- Types of Bias in Research | Definition & Examples
- There's More to AI Bias Than Biased Data, NIST Report Highlights
- The 6 most common types of bias when working with data
- 8 types of data bias that can wreck your machine learning models - Statice
- Data-Driven? Think again
- Overcoming confirmation bias during COVID-19
- AI Bias Causes Problems for Underrepresented Groups
Healthcare is Inherently Biased was originally published in Towards Data Science on Medium, where people are continuing the conversation by highlighting and responding to this story.
from Towards Data Science - Medium
https://towardsdatascience.com/healthcare-is-inherently-biased-b60bf00d4af7?source=rss----7f60cf5620c9---4
via RiYo Analytics
No comments