https://ift.tt/3odoGY9 Leveraging advances in technology and data science to measurably improve public health outcomes for at-risk people i...
Leveraging advances in technology and data science to measurably improve public health outcomes for at-risk people in low- to middle-income countries
Machine learning is one of the hottest topics in health tech today — in almost any field. In this post we’ll be looking at a much less conventional application of 21st century data science. The question we asked ourselves was, “How can we leverage advances in technology and data science to measurably improve public health outcomes for at-risk people in low to middle income countries (LMICs) around the world?”.
Here’s some of the approaches we’re using at PD to tackle this and other public health questions in Sub-Saharan Africa and beyond. We’ll use HIV treatment, one of PD’s main focus areas, to illustrate the approach.
Predictive vs Explanatory Modelling
In our R&D work as a data science implementer, we think of our projects as taking one of two primary routes; predictive or explanatory modelling. Both start off with a dedicated data discovery phase which often informs which route to focus on. It’s also important to note that whichever primary route is collectively agreed upon, both are pursued — and feed into each other.
This is probably a good time to introduce Thabo, our fictional patient case-study subject. Thabo is a 22 year old male, who recently completed his undergraduate degree, and is currently unemployed and looking for a junior position as a medical research assistant before continuing to postgraduate studies. Thabo has also been HIV+ since birth, and virally suppressed his entire life.
Traditional public health theory tells us that Thabo is a young unemployed man, and hence at a significantly higher risk of having an interruption in treatment (IIT) — i.e. missing check-in appointments and going off his antiretroviral (ART) meds for a while, perhaps even a long time. This is a considerable problem for healthcare programs and people living with HIV (PLHIV) since those who disengage from care can develop drug resistance and may need to have costly changes to their regimens.
The clinic that Thabo visits for check-ups and to collect his medication is run by a PD partner, they’re currently conducting user testing of tools that PD developed with the support of the partner to optimise patient retention. After the healthcare worker finishes their standard visit protocol, they explain the predictive tool to Thabo, he agrees, and over the next three minutes they enter the necessary information.
The predictive tool at this clinic is either an app on a tablet (Figure 1 below) or a paper scorecard (Figure 2 below), both of which are machine-learning based tools that output a score approximating the risk of Thabo being late for his next visit by more than 28 days (have an IIT).
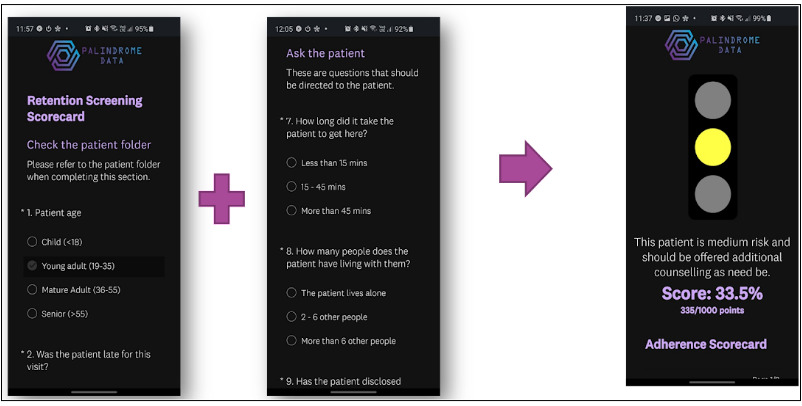
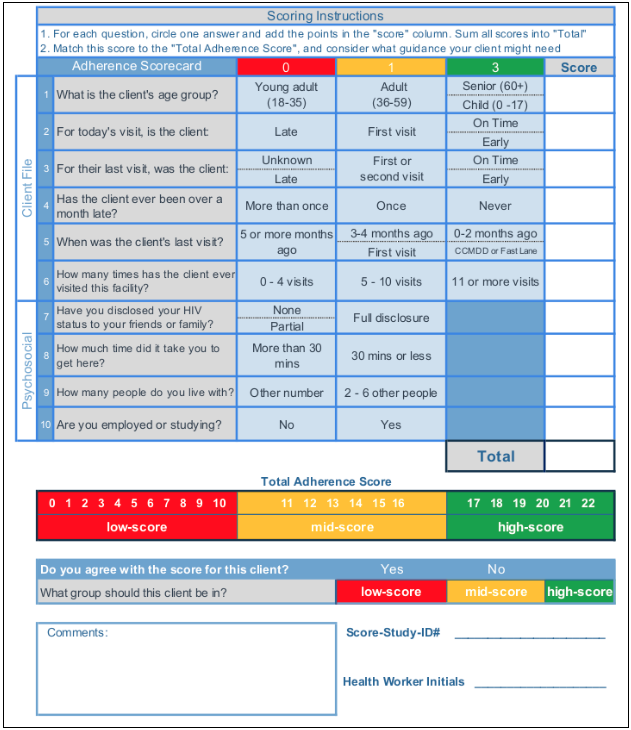
The predictive tool outputs a score based on Thabo’s information, which classifies him as being at an intermediate risk of having an IIT. The healthcare worker has known Thabo for years and knows that the intermediate risk score is a result of the complex happenings of his life. As an additional check, the healthcare worker refers back to Thabo’s file to view his archetype classification, which brings us to explanatory modelling.
Explanatory modelling uses a range of ML techniques, including predictive algorithms, to analyse a population with the end goal of optimising our understanding of population dynamics, groupings, and patterns, as opposed to the end goal of pure predictive power. A primary example of explanatory modelling is patient profiling, with the aim of developing patient archetypes. A patient archetype is a generalised construct that captures a sub-population’s key demographic and behavioural traits. The healthcare worker sees that Thabo was recently classified as a ‘prompt & loyal adolescent boy or young male’, meaning that Thabo was classified as belonging to the archetype representing the young male patients who are among the most stable patients with the lowest likelihood of having an IIT, reinforcing the healthcare worker’s understanding of Thabo’s stable risk profile.
After assessing both predictive and explanatory tools’ outputs, the healthcare worker makes an informed decision to keep Thabo on his long-standing treatment plan, and opts to fill in the short feedback section on the tool — indicating that they disagree with the predictive tool’s output and summarily outlining why.
This feedback loop is invaluable and is thoroughly analysed by PD and partners as part of a continuous monitoring and improvement process. For example, the results and feedback from Thabo’s check-up visit, along with all the other’s from the round of user testing may help us to improve the predictive tool’s equity, accuracy and usability.
The broader effects of wide-scale implementation of tools such as these are far reaching:
- It introduces elements of personalised healthcare to public health systems and under-served communities — especially rural communities with high disease burdens.
- It taps into disproportionate cost-savings that result from being able to segment patients by IIT risk which, in turn, allows for much needed re-allocation of resources.
- It often leads to a much better patient experience where more time is spent on those with greater needs, while reducing the friction experienced by low risk patients.
- It allows healthcare workers to proactively provide interventions, while the patient is still in-treatment, to reduce the IIT burden (most interventions are currently only applied retroactively once an adverse event has already occurred - this opens up a whole new suite of intervention opportunities).
Each of the above benefits creates a positive feedback loop that alleviates pressure on public healthcare systems and workers, and re-orients the focus back unto the patients individually.
There are many other applications and opportunities for data science and machine learning to be used for good in the public health space and PD aspires to contribute in ways that will improve patient outcomes, for more like this follow us on Medium, LinkedIn, and Twitter.
Disclaimer: *Thabo* does not reference any real person or entity and is used solely for illustrative purposes in this post.
Machine Learning in Public Health: A Patient’s Journey was originally published in Towards Data Science on Medium, where people are continuing the conversation by highlighting and responding to this story.
from Towards Data Science - Medium https://ift.tt/3oin1Rb
via RiYo Analytics
ليست هناك تعليقات