https://ift.tt/3kwZN7o This is a three part blog series in partnership with Amazon Web Services describing the essential components to buil...
This is a three part blog series in partnership with Amazon Web Services describing the essential components to build, govern, and trust AI systems: People, Process, and Technology. All are required for trusted AI, technology systems that align to our individual, corporate and societal ideals. This first post is focused on making people across organizations successful with building and implementing AI you can trust.
The benefits of AI are immense. Beyond new revenue, and time savings, AI is being deployed to solve critical problems in healthcare, the environment and government. However, these benefits can come with a risk which must be addressed. Let’s not forget AI is ultimately a humanistic affair where people construct, maintain and monitor systems. Despite the publicity, AI does not really create fully automated decisions learned without human intervention as much as augmented decisions enabled by human intervention. As ML technologists, we must ensure that technology is built in a way that supports a diverse and equitable implementation rather than reinforcing historical mistakes or amplifying bias.
DataRobot was founded in 2012 and today is one of the most widely deployed and proven AI platforms in the market, delivering over a trillion predictions for leading companies around the world. From years of partnering with customers across industries, we’ve learned what it takes to build trusted AI that delivers value, with governance to protect and secure your organization. We’ve learned that people, process and technology are critical components to trusted AI systems.
But people are rarely acting individually when undertaking AI, they are usually building AI in the cloud within an organization. Since our founding DataRobot has collaborated with insurance, banking, retail, manufacturing, transportation, government, and even professional sports organizations. We’ve learned over time, the processes, data fluency, ingenuity and risk tolerances vary greatly across and even within industries. Sound processes enable your people to build AI by clearing up ambiguity. Well-constructed operating procedures don’t constrain people, but build confidence and dispel ambiguity so that AI systems align to organizational goals and ethics.
The first step to trusting AI is to focus on the people related to an AI system so the end state decisions are aligned to organizational goals. The second step is to ensure foundational data fluency so that the AI system has a greater chance of being built successfully while also accounting for the diversity of perspectives among stakeholders. We build these steps into the beginning of every engagement to ensure our customers are successful.
At DataRobot, for some of our most sensitive data science efforts, the project starts with an impact assessment to identify stakeholders. This ensures a multi-stakeholder perspective and DataRobot has made it even easier with our new AI Cloud solution. We’ve found that implementing a model requires four general personas to collaborate across the organization. These include:
- AI Innovators: The business leaders that identify and structure a problem for ML
- AI Creators: The data scientists and technical practitioners collecting data and building models to solve for the AI Innovator’s problem or opportunity.
- AI Implementers: The IT organization that must inherit a model, whether ML Engineers, or more generally ML Ops personnel. They are tasked with getting the model into a stable and robust state requiring many personnel from cyber security to architecture.
- AI Consumers: The internal and external parties that consume the model’s output. This may be an internal Model Risk Management or compliance team or even the individual consumer that must rationalize an algorithmic decision applied to them.
Next, we assess and educate. Despite some of the hype around organizational data maturity, the time tested tenets of Total Quality Management (TQM) Systems hold true today. Professor Yoshikazu Tsuda is a pioneer of quality management systems. He was one of the first to document the data maturity of organizations and a table inspired by his framework is shown here.
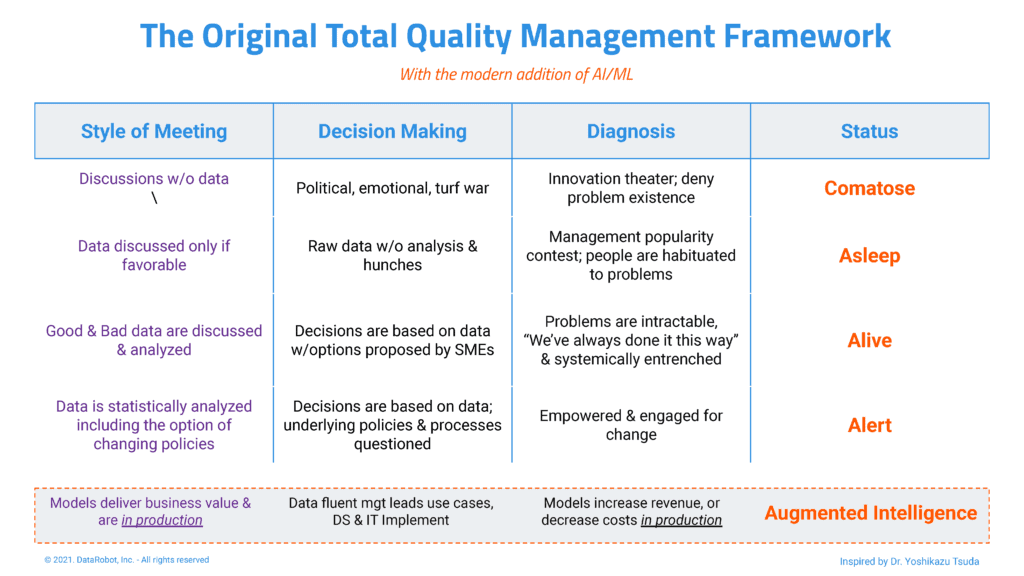
Trusted AI is not a feature, it is a journey. It requires discipline and organization. When education is needed, it also requires change management. So be thoughtful of who is involved in a system, work to increase the diversity of input and then educate the parties for a common understanding. Without focusing on people and their data acumen, a ML project may not solve the problem intended, or worse amplify behavior that is at odds with the organization’s values.
The post How to Build & Govern Trusted AI Systems: People appeared first on DataRobot | AI Cloud.
from Blogs – DataRobot | AI Cloud https://ift.tt/3qu5kQd
via RiYo Analytics
ليست هناك تعليقات