https://ift.tt/3Gq4ZU3 How you build the strongest of data chapters Photo by Andrey Zvyagintsev on Unsplash We live in a time when th...
How you build the strongest of data chapters

We live in a time when the world is advancing at lightning speed. One day we are talking about old companies with billions in revenue, global patents, and distribution, who are “too big to fail”. In the next breath, 9 out of the 10 highest valued companies in the world are all digital, with ex-moonshots that all barely had a decade before reaching the moon. So today we all truly know that, to succeed, the key is called digital innovation and data disruption. And, yes, we also know that data is “the new oil”. But turning off the BS generator and instead focusing on how to realize this for real, how do you go about really succeeding in this data disruption? The slightly less magical answer is that one of the prerequisites is the quality of the data itself, and the architecture and platforms to be able to manage, process and develop the data products. The more dynamic answer is that until the machines are completely taken over from mankind (which does not have to be too far away), the most suitable setup of people is required to fully realize this potential. In this article, we will cover exactly how to build the very strongest of such a data chapter, on what they should be based, what roles are needed, what they need to know, how they should be acquired and be organized to enable true data disruption. Bon Appétit.
1. THE STRATEGIC GOAL
1.1. The digital trend
It is now several years since data was proclaimed as the new oil in the world, then as one of, perhaps the most important, KSF for the last decade’s global unicorns, decacorns and hectocorns when digitally disrupting all traditional companies — operating in all old industries now creatively crushed by everything from SoMe, AdTech, MarTech, AgriTech, FoodTech, FinTech, InsurTech, EdTech, CleanTech, BioTech, MedTech etc. The conditions for this disruption have always been about emerging technologies such as AR and metaverse, blockchain and, above all, AI and ML.
Exactly all this is controlled by the quality and the real-time fast processing of data. All to the extent that since 2020 it has now led to a climax in how the leader of the world’s fastest growing, and (so far) second strongest, digital nation, President Jinping, last year proclaimed data as “the fifth factor of production” — to equate with labor, land, capital, and technology.
1.2. The pivoted value
In line with this, many people today sit in companies that go from having several of the other production factors as the most important core in the company, to developing companies in the most serious terms that aim to, if not today, then at least tomorrow have data as their core asset .
One of the strategic keys to making this a reality is that we are starting to handle Data-as-a-Product (DaaP). I.e., not as a side effect of a multitude of other functions, but as a product with a value in itself, in the extreme cases not only as enabler of data-driven decisions, services and products, but also purely concrete intrinsic value and even business value.
Having a digital unit that creates all the conditions required for a company to have data as a core asset, or at least DaaP, is the very precondition for a company not only to be able to survive on a future market rebooted by creative destruction but take a leading position by being the one who disrupts instead of being disrupted.
In this article, we will address the prerequisites for the success of data disruption, where we first look at how the company’s digital strategy translates into data strategy, data architecture, and suitable data platforms, followed by relevant data squad to manage these platforms, and fully realize the potential of the strategy developed.
2. THE STRATEGIC MEANS
To succeed, several things will be required, not only a) a well-organized data setup, with decom legacy, secured data sources and cleansed data, but both b) a modern data strategy with aligned architecture, and suitable technical and organizational platforms adapted to this architecture, and c) a data squad that both produces the right data, ensures data quality, and transforms the generated data into more data-driven business results. Below, we will assume that the actual cleansing work has been done and treat the latter two more strategically of these issues.
2.1. Digital strategy
After a company has agreed on its vision and the strategy that will realize this vision, it is in digital language the data strategy, data architecture and data flows that constitutes the digitally related translation of the strategy into its data blueprint.
Neither the old 5-year strategic plans or more evolutionary approaches are viable in most industries today, where a more dramatically disruptive vision is often required that it renders a total transformation of its core business from analog technology offline, to digital technology online. Having worked with digital strategy and innovation with a three-digit number of companies on four continents, I see few boards and c-level teams that today don’t know that in a year or so they will have lost billions in revenue from business as usual (BAU). And that this BAU needs to be replaced by new business innovations that few truly identified what it is about — only that if you do not take a position on this unbroken ground, someone else will.
To help achieve this future and often unknown digital Shangri-La, it is thus nothing more than an equally dramatic strategy required to succeed. The up-or-out situation that most old traditional markets have been exposed to, can then never be allowed to stop at digital development to create leverage in existing assets, but must also be about creating growth of completely new businesses — in the more dramatic cases to reinvent whole industries and, thus, pivot whole strategies and reboot of operations.
Quite simply, based on a more innovative strategy that not only looks at how to battle today’s but also tomorrow’s competitors, make sure to dare to invent real moonshots that satisfy not only existing customers ‘existing needs, but also tomorrow’s customers’ latent needs.

On the positive side, in addition to general digitalization, there are several emerging technologies — e.g., AI, AR and blockchain etc., aka EmTech), with the potential to help the company execute on this more offensive disruption. If this is translated into the digital strategy for a company, it will mean an aggressive focus on tackling the challenges posed by external pressure and internal conditions, which includes customer strategy, content strategy, channel strategy and data strategy. Below we will handle the latter and, I argue, during the 2020:s the deadliest crucial one.
2.2. Data architecture
If the digital strategy is to be translated into how the company’s architecture should be built to be able to handle the challenges, then it is about an at least as dramatic pivot in the digital architecture, lineup of platforms and organization that are adapted to this particular strategy.
Not knowing what exact architecture, you and your company will have in the future, there is usually at least some guidance given a look at the digital strategy — which in a digitally disruptive market often is translated into a DaaP-based data strategy to succeed.
Slightly depending on your contextual conditions, architecturally such a DaaP strategy should at least take into account any of the modularly built hybrids that lie somewhere in the spectra between a decentralized data mesh and monolithic datalake — ie. moves in the direction of a domain-driven architecture for maximum data quality and innovation, while the synergy between different channels is ensured by a federated governance, etc.
In terms of technical platform, this would mean a dynamic system that offers both a local ELT pipeline as self-service system, which is ensured by a central platform of standards securing interoperability, supplemented with various data science tools for AI/ML.
The organization suitable for such a solution would be a balance between a local stewardship with data owners, which for standardization, interoperability and innovation is ensured by data engineers and data scientists hubbed centrally but allocated locally. More about this below.
3. DATA SQUAD— THE THREE MOST CRUCIAL ROLES
As we have seen above, one of the fundamental prerequisites for data disruption is the quality of the data itself, while another is the architecture and platforms to be able to ingest, store, manage and process this data. The main outlines for this that we arrived at above can never constitute more than a tentative hypothesis deduced from the digital strategy — to be validated, falsified, or tweaked during the progress to a more granular version of a data architecture and organizational model.
But having said that, given that a company is through the above strategic deduction and the development of the architecture, lineup of platforms and organization that tend to be best suited for such a strategy, we inevitably end up at the question of how we in a digitally disrupted industry should man up to fully realize the data conditions provided by architecture, platforms, and organizational model. Cause until machines are completely taking over from mankind (which does not have to be that far away), the most suitable setup of people will always be required to fully realize the built-up potential.
Below these questions are divided into what roles are needed, what will be their tasks and what skills they should be expected to have, followed by questions about quantity and cost, and how such as squad should be managed and allocated in the best way. These issues are dealt with in turn below.
But first to the roles, where at least three data related roles are needed in a company to be able to develop data to a level that means that data could really become a “core asset” in a company.

3.1. Data engineers
The first of these is the purely technical, i.e., the data engineers who ensure that the right data can be retrieved. This can be about building and managing the data infrastructure available at the company, incl. to select databases, hardware, or cloud provider, calculate the type and number of servers and what software is needed for the database and servers. They manage the data storage, use, and monitor how it works and who uses it. And, as a baton, can extract data or issue access to certain data that is passed on for analysis or processing in the next stage of the value stream.
To succeed in this, the data engineers need to have a broad knowledge about computer-related infrastructure, partly about hardware for both storage and use, partly about software for databases, calculation, installation, scalability, etc. The background is often computer science or computer technology or another IT-subject related to infrastructure
3.2. Data Scientists
The second role is the purely “analytical”, in the form of the data scientists who convert the data from raw material to finished product, traditionally by running experiments, extracting, and cleansing data, analyzing it, and communicating the results to various stakeholders inside or outside the organization .
This is only partly about historical analysis, with slightly simpler parts in the form of descriptive data (“reports” and dashboards “) and purely diagnostic analyzes (exploratory) as more data-driven insights for decisions (can be handled both by data scientists, but also by analysts, sometimes even relative juniors). For the company with DaaP ambitions, the challenges are instead primarily two different parts of a more futuristic nature:
1) More automatic prediction of probable behavior from parties such as customers, employees, suppliers, distributors, as well as prescriptive data for which action tends to generate the most favorable response from each party for the company (e.g., “mass-customization” with personalized prediction of NBA/NBO/NBC etc.).
2) Perhaps the most advanced effort will be in terms of the data enabling innovation and product development at the company, as well as generating enriched data that has such value in itself that it should in itself be able to generate concrete revenue for the company.
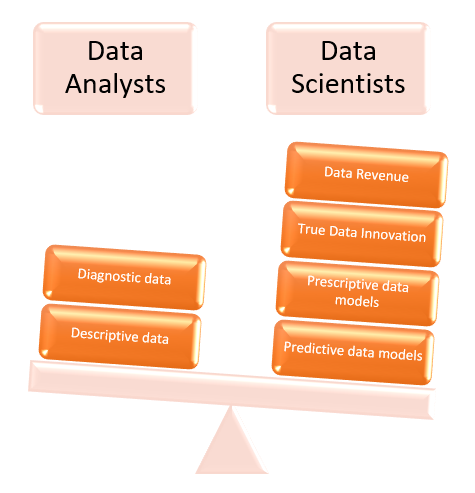
These two latter parts are a job for real stars of data scientists, and to succeed you must be able to handle not only statistical inference but also advanced prediction models — the former preferably with a degree in statistics but otherwise supplemented with online courses, the latter preferably with a degree in programming suitable for ML (e.g., R/Python), if not supplemented with online courses.
You must thus master advanced data analysis while at the same time be sufficiently tech savvy to be able to extract data from databases (e.g. SQL), clean them, statistically analyze and predict them, and communicate the result (incl. visualization and communication for management and “normal” people) The same applies at the very beginning of the process, with an ability for dialogue in which stakeholder challenges are translated into concrete statistical models and experiments.
As data science is still a relatively “young field” without total rigor on the academic side (there are comparably few but at least growing numbers of strict educations in specific “data science”, but above all they are so new that they have not had time to deliver an impressive number of graduates yet), there are generally two different types of backgrounds.
1) A solid statistician who learned some programming.
2) A solid programmer who learned some statistics.
I have personally met awesome data scientists in both of these categories, and I myself — who as a member of the former group may be a bit biased — might have identified somewhat more examples of stars (= more self-propelled) among the former compared to the latter (who I have more often experienced need to be winged by an analyst or strategist to avoid pitfalls and be able to form a good bridge for stakeholders). Still, I have found a big bunch of real stars also in the latter area (not infrequently when they have a more solid addition of inference or supplemented with something MBA-ish). And as small as the supply is compared to the demand today (see more below), we must be grateful that both exist 😊

3.3. Data owners
The third role is perhaps the most “interesting”, where the hybrid of data architecture that the digital strategy in a predicted disruptive market indicates the need for — i.e., the combination of the best with the monolithic stability at the federated level and the data meshy dynamics at the domain level — creates a completely different need for responsibility for the data quality in the actual domains.
This means launching a type of domain-related “data owner”, something that is rarely found in offline companies, and which may very well mean a leapfrog in the development towards DaaP. That is, a person who, in addition to his regular work in each domain, ensures that we live up to regulatory requirements and the company’s policy regarding GDPR/CCPA etc. in a dynamic way — where you have an information classification with different security values for different types of data, and taking different measures based on that classification.
But above all that you as a data owner shoulder the responsibility for the quality of the data generated within the domain, partly in terms of cleansing and any DQM required for data ingested and stored in the domain, and partly that it is handled correctly in any ELT mode locally — and, in the most qualified mode, the role of co-creating “data enabled products” as a bridge between the company’s stakeholders and various experts from the data squad.
In terms of time, this regards only in exceptional cases — i.e., in really large organizations with large domains — a full-time job, but a responsibility that is assigned to some role in the domains. In terms of competence, it is also not necessary — or perhaps even desirable — to have either a statistician or an engineer for this function, but someone who understands the business in each domain, and is sufficiently gifted analytically to not be afraid when it comes to data.
Instead, the major advantage will the understanding how data relates to the business, when it does not, and when there is something wrong with it. Most of all, in addition to business understanding, it may be about responsibility, the understanding of the importance of data, and ensuring its relevance and quality of each data type.
4. YOUR NEW DATA CHAPTER 2022 — THE PROCESSES FOR RECRUITMENT AND LEADERSHIP
When it comes to what number that a company will need in the three different roles it is dependent on the maturity of the company, but three things I would like to claim are the most important when getting started is the agile appointment, the open-minded recruitment, and the two-dimensional organization.
4.1. Agile appointment
Firstly, there are few reasons to act with a big bang, instead of starting off with a smaller number, not impossible including som runner up leadership, and then be well prepared to switch up when you start hearing the first complaints about how full their agendas are. It is not impossible that even if the end game aims for a full-fledged data squad, it may at first be about a few data engineers with a squad leader for the federated infrastructure centrally while allocating some part-time roles to a smaller set of domains in their first iterations.
At the same time, the data engineer’s role is more about infrastructure and its role as a support function (i.e., once the basic need is in place, you do not get more business value for taking in 10 than taking in 3). What is most commercially crucial is instead the number and quality of data scientists. But here too, regardless of end game, you can also start small-scale with a lead, and then increase for different functions — where someone may allocated half a time to a few domains, and another is dedicated to more central data innovation. But here the equation is commercially simpler. With 10 awesome data scientists, you will (when infrastructure and data quality are really in place) contribute 10 times more value.
4.2. Open Minded Acquisition
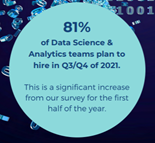
The second important thing to understand is that this is a different type of breed. You are not the only one in the world who understood that data needs to become your company’s core asset, and that the high-quality data pros are the alchemists needed to reap the true benefits out of these assets.
This is of course a general issue. In general 90% of executives are experiencing a skill gap in their organizations, and most of all we see it within digital roles, where over the next decade the G20 countries can miss out on as much as $11.5 trillion of cumulative growth promised by intelligent technologies if they cannot meet future skills demand. Of course, that shows in the wages and demands from our digitally skilled go-getters. And, apart from health and cleantech and information security, the biggest demand of them all will be for statisticians and data scientists.
So not only are there some specific things to keep in mind when it comes to recruiting digital stars in general, but for data scientists in particular it is clearly the seller’s market, and the role is so “sexy” that prices even exploded offshore. The data scientists, but to some extent also data engineers, are simply extremely highly sought-after critical resources on the market.
This means several things. Partly that you need to have something “sexy” for them, or us, to bite into, not only as a company but also as a data-driven ambition and data-innovation challenge. Partly that they do cost — I’ve had newly graduated stars from the tech university with zero experience that I, in comparison with average salary for experienced digital abilities, had to pay + $40K + bonus for.
Partly that for not only economic but also quality reasons you often have to look outside the digital hubs in the western world — apart from new graduates, all my stars (WITH experience) have been immigrated experts from China, Vietnam, Korea, or Iran (+ 20–40K v/s digitals). Partly that you can, or should, think in terms of offshore (over the years, apart from India, I’ve had mine in Vietnam, totally -! — God-blessed, and in terms of results even slightly better than my onshore).
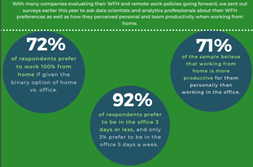
It also means that one does not necessarily, especially not in a post-covid situation on the way to metaverse may require “office work”. Instead, the job is often done independent of the place and if you are to succeed in recruiting the real stars (perhaps without too much room to pay the real star salaries), then you must be a bit liberal here too. In a close case, with about ten onshore data scientists, not one Is locally recruited and even the majority of the onshore occasionally work from Brazil, Croatia, Spain or from home.
Finally, this means that based on the above, one must accept that corporate language, at least for data, must be English (if it is not already). You come way longer with an imported star with a perfect match, than any old retired local analyst from the research industry, which is what you get, at most, for the same price. And even if you step up in price, for the matter of competitive advantage you come far further with three offshore stars at a distance, than what you get for an expensive local western consultant for the same price.
4.3. Two-dimensional organization
The third important thing is how all these roles should be organized. This also naturally follows from the data master architecture that is produced in the company. With the above continuum as a working hypothesis for a company taking its first steps into disruption, whether proactively or reactively, I would say that on the one hand it is highly probable that the company would or should continue in line with DDD, where the company’s data scientists would then make their very best effort out at the domains.
On the other hand, it is also true that data scientists are often people who can, and need to be, a little different (some might call them/us “odd”), that there are not always many others in an organization who understand them or are interested in the same things to talk about on the coffee break. This means that in my world there is a clear both social and cognitive value in organizationally chaptering data scientists centrally with both leadership and interaction that understands them and can help challenge and develop them, but with allocation and “dotted” line out to the domains for cross-fertilization with the business understanding that generates the opportunity to make a data difference “for real”.
5. CONCLUDING SUMMARY
With an overall vision that in a disruptive market cannot be allowed to be anything other than dramatically innovative, as well as a digital strategy that for the same reason needs to have data disruption as its core, the topic of the article cannot be seen as anything less than deadly crucial for the future of any company acting within such an industry.
Without knowing the exact data architecture of your company, with reference to the digital strategy needed for a company operating in such a disruptive market, at least some indication is given as to which direction such an architecture should take. Slightly simplified, more specifically one of all the DaaP-inspired hybrids that can be found in the continuum between the dynamics that a domain-driven data mesh can entail, and the stability and ensured interoperability that a federated data infrastructure can contribute with.
This has implications not only for the most appropriate technical data platform, but also which organization model will be the best tool for succeeding with such a disruptive digital strategy. Simplified this will regard a two-dimensional organizational model where you one hand supports a company’s direction towards DDD for dynamics, with a local stewardship that on the other hand is chaptered centrally to ensure synergies in knowledge and job satisfaction.
The three roles that this organizational model should be filled with regards a small squad of data engineers who on the federation level secures that the right standards of data emerge. To this we add a slightly bigger number of data scientists who are responsible for refining the raw material of standardized data into data-driven insights, data enabled innovations and products and data as a business in itself. Finally, we combine this with data owners who ensure business-relevant data quality at the domain level.
To succeed in the recruitment of the former two, based on the extreme hunt for talents in the market, it can be argued that — in addition to the above-mentioned two-dimensional organizational model — it is highly worthwhile to consider the possibilities for an agile appointment instead of the big bang, and a more open-minded recruitment in terms of both offshore, immigration and remote work possibilities also for onshore.
Having done all this, you’ve just triumphed with the building block that is perhaps the last needed to succeed in what constitutes the most important prerequisite in most markets of tomorrow, ie: data disruption.
So, break a leg, and see you on the other side 😊
Rufus Lidman, Fil. Lic.
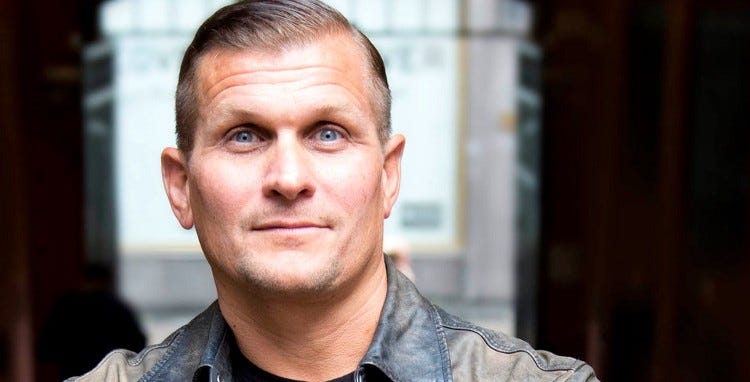
Lidman is a renowned digital innovator and data disruptor, serial social entrepreneur and top tech influencer with 50,000 followers. With dual degrees, PhD-studies complemented with data science as platform, he has been founder of IAB, digital advisor for WFA, recognized speaker with over 300 lectures, and has had assignments within digital strategy and innovation for over 100 companies such as Samsung, IKEA, Mercedes, Electrolux, PwC etc. As a digital entrepreneur he has run half a dozen ventures with 2–3 ok exits, incl. sites with millions of visits and some of the world’s largest apps in its areas with over 15 million downloads. As his latest ventures he co-founded the leading talent acquisition company for digital talents in Sweden, Digitalenta, and founded EdTech PTE Ltd in Singapore, using leading technology to reinvent learning for needy in emerging markets. To that he adds 4 published books and the world’s largest learning app in digital strategy loved by 200.000 people in 165 countries.
The Prerequisites for Data Disruption was originally published in Towards Data Science on Medium, where people are continuing the conversation by highlighting and responding to this story.
from Towards Data Science - Medium https://ift.tt/3pJ7T0e
via RiYo Analytics
No comments