https://ift.tt/3nrkkej Frequentists want to repeat the experiment Photo source Although both have existed since at least the eighteenth...
Frequentists want to repeat the experiment
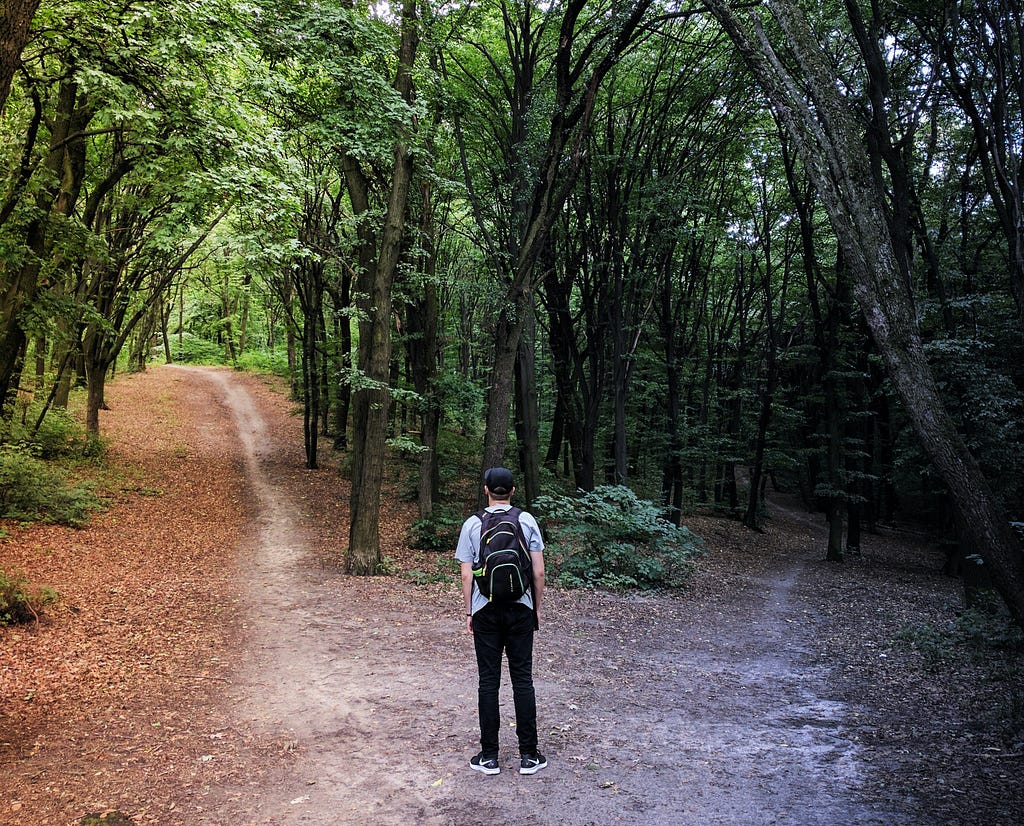
Although both have existed since at least the eighteenth century, frequentist statistics were far more popular than Bayesian statistics for most of the twentieth century. Over the last few decades, there has been a debate — I’ll stop short of saying feud — over the merits of one versus the other. I don’t want to fan the flames of the debate, but the two schools of statistics are mentioned in conversation and in literature often enough that it’s helpful to have a decent idea of what they’re about.
The primary difference between the two is a theoretically interpretive one that does have an impact on how some statistical models work. In frequentist statistics, the concept of confidence in a result is a measure of how often you’d expect to get the same result if you repeated the experiment and analysis many times. A 95% confidence indicates that in 95% of the replicates of the experiment, you’d draw the same conclusion. The term frequentist stems from the notion that statistical conclusions are made based on the expected frequency, out of many repetitions, of a particular event happening.
Bayesian statistics holds more closely to the concept of probability. Results from Bayesian statistical inference, instead of having a frequentist confidence, are usually described using probability distributions. In addition, Bayesian probabilities can be described intuitively as a degree of belief that a random event is going to happen. This is in contrast with frequentist probability, which describes probability as a relative frequency of certain random events happening in an infinite series of such events.
To be honest, for many statistical tasks it doesn’t make a difference whether you use a frequentist or Bayesian approach. Common linear regression is one of them. Both approaches give the same result if you apply them in the most common way. But there are some differences between the two approaches that result in some practical differences, and I’ll discuss those here.
Disclaimer: I’m primarily a Bayesian, but I’m not so one-sided as to say that frequentist approaches are bad or inferior. Mainly I feel that the most important factor in deciding on an approach is understanding what assumptions each of them carries implicitly. As long as you understand the assumptions and feel they’re suitable, either approach can be useful.
Prior distributions
Bayesian statistics and inference require that you hold a prior belief about the values of the model parameters. This prior belief should technically be formulated before you begin analyzing your main data set. But basing your prior belief on your data is part of a technique called empirical Bayes, which can be useful but is frowned on in some circles.
A prior belief can be as simple as “I think this parameter is pretty close to zero, give or take one or two,” which can be translated formally into a normal distribution or another appropriate distribution. In most cases, it’s possible to create non-informative (or flat) priors, which are designed to tell your statistical model “I don’t know,” in a rigorous sense. In any case, a prior belief must be codified into a probability distribution that becomes part of the statistical model. In the microarray protocol comparison example from earlier in this chapter, the hyper-parameters that I described are the parameters of the prior distributions for some of the model parameters.
Some frequentist statisticians take exception to the necessity of formulating such a prior distribution. Apparently they think that you shouldn’t have to formulate a prior belief if you know absolutely nothing about the model’s parameter values prior to seeing the data. I’m tempted to agree with them, but the existence in most cases of non-informative prior distributions allows Bayesians to sidestep the requirement for a prior distribution by making it irrelevant. In addition, the frequentist statistics concept of having no prior belief, if you attempted to formalize it, would look a lot like a non-informative prior in Bayesian statistics. You might conclude that frequentist methods often have an implied prior distribution that isn’t denoted explicitly. With this, I don’t mean to say that frequentists are wrong and that Bayesian methods are better; instead, I intend to illustrate how the two approaches can be quite similar and to debunk the notion that the requirement of having a prior belief is somehow a disadvantage.
Updating with new data
I’ve explained how the existence of a prior distribution in Bayesian statistics isn’t a disadvantage, because most of the time you can use a non-informative prior. Now I’ll explain how priors are not only not bad but also good.
One of most commonly cited differences between frequentist and Bayesian statistics, along with “You have to have a prior,” is “You can update your models with new data without having to include the old data as well.” The way to accomplish this is quite simple in a Bayesian framework.
Let’s assume that a while back you had a statistical model, and you received your first batch of data. You did a Bayesian statistical analysis and fit your model using non-informative priors. The result of fitting a Bayesian model is a set of parameter distributions called posterior distributions because they’re formed after the data has been incorporated into the model. Prior distributions represent what you believe before you let the model see the data, and posterior distributions are the new beliefs based on your prior beliefs, plus the data that the model saw.
Now you’re getting more data. Instead of digging up the old data and refitting the model to all the data at once, using the old non-informative priors you can take the posterior distributions based on the first set of data and use those as your prior distributions for fitting the model to the second set of data. If the size of your data sets or computational power is a concern, then this technique of Bayesian updating can save considerable time and effort.
Today, with so many real-time analytics services under development, Bayesian updating provides a way to analyze large quantities of data on the fly, without having to go back and reexamine all the past data every time you want a new set of results.
Propagating uncertainty
Of all the differences between frequentist and Bayesian statistics, I like this one the most, though I haven’t heard it mentioned that often. In short, because Bayesian statistics holds close the notion of probability — it begins with a prior probability distribution and ends with a posterior probability distribution — it allows uncertainty to propagate through quantities in the model, from old data sets into new ones and from data sets all the way into conclusions.
I’ve mentioned several times in this book that I’m a big fan of admitting when uncertainty exists and keeping track of it. By promoting probability distributions to first-class citizens, as Bayesian statistics does, each piece of the model can carry its own uncertainty with it, and if you continue to use it properly, you won’t find yourself being overconfident in the results and therefore drawing false conclusions.
My favorite of the few academic papers that I published in the field of bioinformatics emphasizes this exact concept. The main finding of that paper, called “Improved Inference of Gene Regulatory Networks through Integrated Bayesian Clustering and Dynamic Modeling of Time-Course Expression Data” (PloS ONE, 2013) — the title rolls off the tongue, doesn’t it? — showed how high technical variances in gene expression measurements can be propagated from the data, through the Bayesian model, and into the results, giving a more accurate characterization of which genes interact with which others. Most prior work on the same topic completely ignored the technical variances and assumed that each gene’s expression level was merely the average of the values from the technical replicates. Frankly, I found this absurd, and so I set out to rectify it. I may not quite have succeeded in that goal, as implied by the paper having so few citations, but I think it’s a perfect, real-life example of how admitting and propagating uncertainty in statistical analysis leads to better results. Also, I named the algorithm I presented in the paper BAyesian Clustering Over Networks, also known as BACON, so I have that going for me.
An excerpt from Think Like a Data Scientist by Brian Godsey.
Brian Godsey, Ph.D., is a mathematician, entrepreneur, investor, and data scientist, whose book Think Like a Data Scientist is available in print and eBook now. — briangodsey.com
For more, download the free first chapter of Think Like a Data Scientist and see this Slideshare presentation for more info and a discount code.
The Bayesians Have Won Data Science was originally published in Towards Data Science on Medium, where people are continuing the conversation by highlighting and responding to this story.
from Towards Data Science - Medium https://ift.tt/3EjNumF
via RiYo Analytics
ليست هناك تعليقات