https://ift.tt/3bkKDxj How to Slash the Error of COVID-19 Tests with a Sharpie: Why Accuracy Is Not Just a Percent Value Why accuracy is n...
How to Slash the Error of COVID-19 Tests with a Sharpie: Why Accuracy Is Not Just a Percent Value
Why accuracy is not just a percent value
In this article I will explore a basic concept in Data Science — accuracy — and demonstrate how a common lack of understanding of this fundamental concept can lead to some very wrong conclusions. Specifically, we will be looking into the accuracy of Lateral Flow Covid-19 tests and find that it is lower than the accuracy of a test anyone can make at home with a sharpie and a piece of cardboard. We will see that accuracy as a percent value is not a useful measure to evaluate such tests. The conclusions are not limited to medical testing, because from a conceptual viewpoint tests are simply classifiers. Just like Data Science models they predict a class (presence of a virus) based on limited information (sample).
Accuracy of Lateral Flow tests
First, we need to find the accuracy of Lateral Flow tests for Covid-19. The answer can be found in a scientific paper “Rapid, point-of-care antigen and molecular-based tests for diagnosis of SARS-CoV-2 infection (Review)” — a 400 page review of 58 individual studies estimating accuracy of Covid-19 tests. We will focus on individuals without symptoms only to keep things simple. The answer lies on page 6 and is not as straightforward as you may think.
The scientific paper does not actually provide accuracy directly, but instead sensitivity and specificity — two values that are unpacked in Figure 1 with the values from the paper filled in Figure 2. Sensitivity is just a different term for the True Positive Rate, i.e. how many people with the virus will receive a positive test result. Similarly, specificity is just a different term for the True Negative Rate, i.e. how many people withOUT the virus will receive negative test result. Using sensitivity and specificity we can construct a confusion matrix a description of how likely to be correctly classified people with/without Covid-19 are:
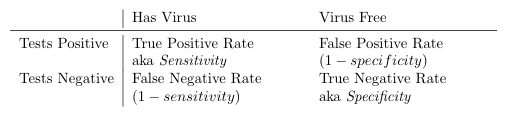

Now that we know what are the False Positive and False Negative rates we can calculate the accuracy. To estimate accuracy we need to consider how many people actually have the virus. Without considering the prevalence of the virus the numbers in the confusion matrix don’t really represent what will happen when we use the test in real world. According to ONS [1] this is currently 1 in 260 individuals.

Accuracy is the probability of obtaining the correct result, regardless of whether it is positive or negative. Using the expected confusion probabilities from Figure 3 we arrive to 98.7%, which seems impressive indeed! However, I took it upon myself to push this number even further, and developed my own Covid-19 test with even higher accuracy. I will call my new testing method the Cardboard test.
Accuracy of Cardboard test
The Covid-19 test I have developed, pictured in Figure 4 consists of a piece of cardboard with “NEGATIVE” written on it with a sharpie. The advantages of such a test are huge — the cost of manufacturing is very low, it is reusable and the result is immediately available.
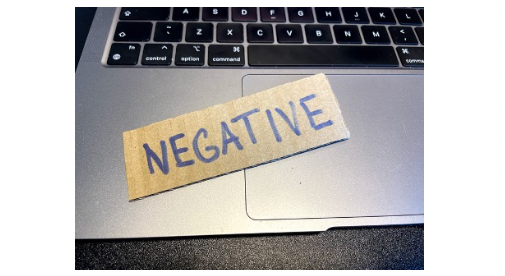
While this may seem ridiculous, please bare with me to calculate the accuracy of this test. Let’s start with the confusion matrix:

Following is the confusion expectancy matrix to account for the fact that only 1 in 260 people in UK have Covid-19 at the moment.

As accuracy is the probability of obtaining a correct result whether positive or negative, using the expected confusion probabilities from Figure 6 we arrive to an accuracy of 99.6%. A notable improvement compared to 98.7% accuracy of the Lateral Flow tests.
This value implies the amount of errors made by the Cardboard test, when used on asymptomatic individuals in the UK at the moment, will be 5 times lower than the amount of errors made by Lateral Flow tests currently offered. This may sound strange, but is a simple result of naive interpretation of accuracy, the public health authorities luckily know better than to look at the accuracy alone and therefore are not tempted to switch testing to my Cardboard tests however cost-saving that might be. The reason why the cardboard test is not used is that different types of errors have different implications to public health.
Receiving a False Positive test result is a nuisance, pushing someone needlessly into isolation. A False Negative means that someone with Covid-19 will be walking around spreading it, putting lives at risk and increasing strain on health services. Clearly these two outcomes have a completely different impact and should not be considered equal. This is the reason why good decision-makers consider Expected Cost of their decisions rather than accuracy of a test or prediction alone. Cost is a technical term for a negative impact of a decision-making error. The other side of the coin is called Expected Utility and is often used in business contexts — to evaluate the benefit your decisions are expected to bring, in which case you consider the True Positive and Negative Rates.
Expected cost is very easy to figure out once you have obtained the expected confusion matrix and are able to attach cost to the different types of error. Quantifying the cost of errors is not always easy and this is one of such cases. However, for the purpose of this exercise we will simply assign a False Positive result a cost of 1 arbitrary unit. We will make the assumption that a False Negative is 10 times as costly as a False Positive, while correct results (True Positive or True Negative) have 0 cost associated with them. Multiplying the appropriate cells in the confusion expectancy matrix yields the following result:
Figure 7 clearly shows that the expected impact of using the real and cardboard tests is quite different, with the cardboard test’s errors resulting in more than double cost than the real result. This leads to the reasonable conclusion that the real test is a better tool to tackle the pandemic than the cardboard test developed by myself, despite its higher accuracy.
This article demonstrated how percent accuracy is not a useful measure for evaluating tests, models or any other classification tool that impact the real world. To properly evaluate classification tools it is necessary to look at sensitivity and specificity, construct a confusion matrix, expected confusion, and expected cost of the decisions that will be made based on the classification. It may seem like a lot to ask, but without doing so we may end up using a piece of cardboard instead of real Covid-19 tests to tackle a pandemic.
References
[1] Coronavirus (COVID-19) Infection Survey, UK: 2 July 2021.https://www.ons.gov.uk/ peo-plepopulationandcommunity/healthandsocialcare/conditionsanddiseases/bulletins/coronavirus-covid19infectionsurveypilot/latest.
[2] Jacqueline Dinnes, Jonathan J Deeks, Sarah Berhane, Melissa Taylor, Ada Adriano, Clare Davenport,Sabine Dittrich, Devy Emperador, Yemisi Takwoingi, Jane Cunningham, et al. Rapid, point-of-careantigen and molecular-based tests for diagnosis of sars-cov-2 infection.Cochrane Database of SystematicReviews, (3), 2021.
How to slash the error of Covid-19 tests with a sharpie was originally published in Towards Data Science on Medium, where people are continuing the conversation by highlighting and responding to this story.
from Towards Data Science - Medium https://ift.tt/3bhjICt
via RiYo Analytics
No comments