https://ift.tt/2Zz2Swi Setting up the infrastructure to drive a culture of effective analysis Photo by Ricardo Gomez Angel on Unsplash ...
Setting up the infrastructure to drive a culture of effective analysis
In a previous post I outlined the drive for a culture of experimentation and the principles of analysis required to entrench this mindset shift across a business. However, it’s time to shift gears — before propelling continuous learning through experimentation, we need to go back to the very foundations of data capture and transformation.
You would think that as such an integral component of product development, there would be more emphasis on integrating experimentation with data infrastructure. Sure, you get the likes of Netflix and LinkedIn leading the way with their own experimentation platforms — but what about the small fish? I want to explore how even smaller companies can overcome the limitations of out-of-the-box experimentation solutions and progress towards building similar in-house platforms.
The old buy vs build adage
Why is it, that for many applications of prescriptive analysis, we’re happy to engineer data from raw ingestion through to transformation — but for experimentation (arguably the crux of combining descriptive and predictive analytics into intelligent decisioning), we’re content with plug and play solutions? To realise the power of integrating experimentation with internal data infrastructure we need to firstly understand the limitations of the optimisation tools that are readily available.
Plugging into readily available experimentation solutions such as Google Optimize or Optimizely has typical out of the box advantages: ease of setup, implementation and insight. Consider, however, these limitations:
- Data inputs— out of the box solutions are built for digital experiments on customers’ experience across websites and apps. Once you include any touchpoints with customers that happen offline (for example, applications converted over the phone), then implementation is not as simple. You can upload offline touchpoints as conversions through API configurations, though this starts to take away from the ease of implementation these solutions offer in the first place;
- Data outputs— existing experimentation platforms thrive on the insights they make available, i.e primarily revolving around (and rightly so) experiment analysis. However, querying the experiment data for other forms of advanced analysis and visualisation is only available if you export the event data from the platform.
Put frankly, with the effort required to set up your experimentation solution with inputs from your other data sources, then to export these data outputs for further analysis — you might as well build experimentation into your own data stack. Not only that — integrating your experiment data with your customer’s data end-to-end doesn’t just benefit your experimentation culture, but enriches your view of your customer overall; enabling more agile analysis and personalisation at scale.
Building the experimentation data infrastructure
So if we are to foster an iterative, agile environment for effective experimentation culture, we need a framework for integrating an experimentation solution into our data infrastructure. Albeit this framework needs to be considered alongside the backend that powers random assignment, running concurrent experiments, and variant retrieval requests — given this has been more extensively explored, I want to focus on the data-related components in this framework. There are five key components:
- Digital analytics measurement plan— mapping out the key user parameters (e.g acquisition sources: are they new or existing customers? Have they been driven by specific marketing channels?) and event touchpoints that occur across your digital landscape. Make sure you are tracking a user ID that can connect the events for an individual user across multiple sessions;
- End-to-end data blueprint — once your measurement plan is implemented, the next step is to connect your digital landscape with customer touchpoints that occur offline, with a person ID* or the like that can be used as a unique key across your whole service blueprint. This will allow you to map out the entire journey for each individual person, enabling a single view of your customer;
- Experiment data model — outlining the data to be collected to understand every experiment and variant a person is exposed to, and whether they converted on both primary and secondary metrics. Then mapping this to the rest of your data blueprint and automating the ingestion and transformation of this data for analysis;
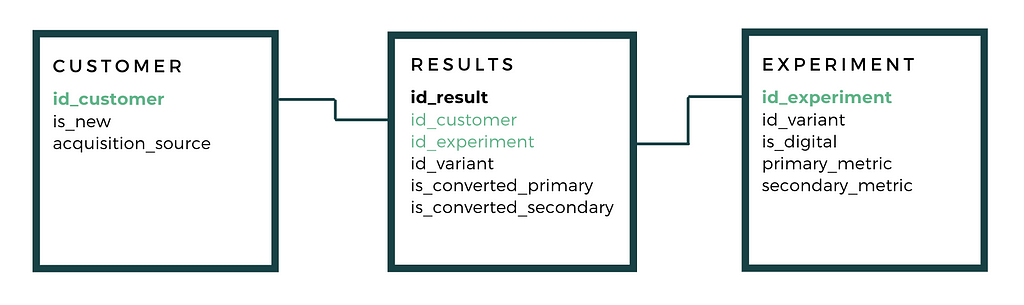
- Frontend experimentation interface— developing a tool that analyses statistically significant difference between your variants. Part of this includes surfacing the principles of Bayesian analysis when sample sizes are lower and statistical significance is harder to achieve. Visualising this analysis effectively is also crucial to driving a culture of experimentation, so that anyone is able to understand the results and take action;
- E2E experimentation strategy — as with any other product, data products are not successful unless they are adapted by their end-users. Training teams on how to use the experimentation interface, and developing and maintaining an ideas registry are all part of fostering momentum around cross-functional experimentation.
Successful experimentation analyses that paves the way for a thriving test and learn culture depends on a framework to integrate your experiment data with the rest of your data infrastructure. Integrating the collection, transformation and analysis of your experiment data with the rest of your customer data ultimately enables experimentation to be engrained into the culture of your organisation, to become pivotal to your product strategy.
- For the purposes of this post, we are dealing with an actual person, i.e with a heartbeat. This could look different if you’re dealing B2B.
Experimentation: Backing It Up was originally published in Towards Data Science on Medium, where people are continuing the conversation by highlighting and responding to this story.
from Towards Data Science - Medium https://ift.tt/3jUrve5
via RiYo Analytics
ليست هناك تعليقات